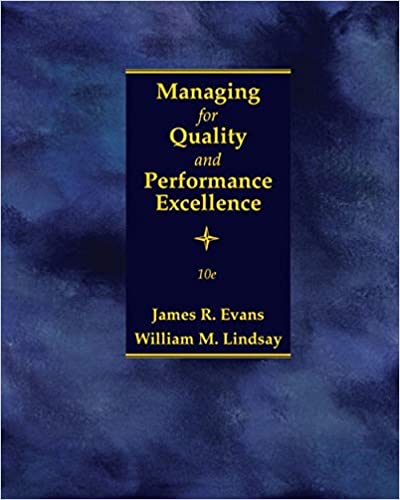
Managing for Quality and Performance Excellence 10th Edition by James Evans ,William Lindsay
Edition 10ISBN: 978-1305662544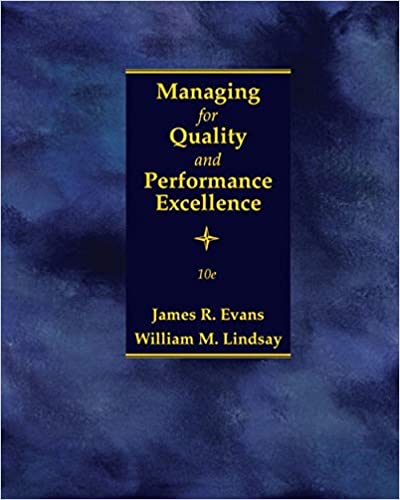
Managing for Quality and Performance Excellence 10th Edition by James Evans ,William Lindsay
Edition 10ISBN: 978-1305662544 Exercise 26
As we noted at the beginning of this chapter, statistics first gained popularity in business and industry in the 1920s and 1930s-principally in production-as a consequence of the development of control charts and other methods for statistical quality control. The demands of fault-free mass production and the systems manufactured for the defense effort during World War II furthered the use of statistical methods. That carried over to business and industry in the years following the war. The major emphases, in addition to helping ensure manufacturing stability, were on checking that incoming materials met specifications and on rejecting bad product. As organizations began to focus more on customer satisfaction and designing quality into products and processes, it became clear that statistical concepts in general and design of experiments in particular could play an important role in helping build and validate quality products. Today, a wide variety of applications of statistics are used in quality. Among them are the following.
Product design and reliability: The major goal of statistics in product design is to help develop the best possible product and to guide its successful transition to manufacturing. This includes involvement in setting design goals, ensuring measurement capability, designing a product that meets or exceeds the goals, validating the design, and transitioning it to manufacturing. For example, statistics can help:
• Determine customer needs and wishes through market-research studies.
• Evaluate the impact of critical variables on product characteristics and assess robustness to variability in manufacturing conditions and product use through designed experiments.
• Assess and improve product reliability through the design and analysis of accelerated test programs. In the past, reliability activities were mainly reactive, dealing principally with responding to unexpected field failures-for example, quantifying the severity of a problem, assessing whether a recall is needed, and, if so, determining what segment of the product population needs to be recalled. Such problems, unfortunately, still arise today. But the main focus is on avoiding them and learning how to build a consistently high-reli-ability product in the first place.
Many of these concepts are discussed further in Chapter 7.
Manufacturing: The major goal of statistics during manufacturing is to help ensure the building of a consistently high-quality product. Typical applications are to help plan and analyze the results of programs to:
• Assess manufacturing capability and stability.
• Evaluate and remove measurement bias and quantify and reduce measurement variability through gauge repeatability and reproducibility studies.
• Remove deviations from target performance and reduce performance variability.
• Monitor the process-often using control charts -to signal significant changes, to identify and remove special causes of variation, and to provide the path to permanent improvement.
These are discussed further in Chapter 8.
The manufacture of discrete parts often calls for the use of different statistical tools from those used in the processing industries. It may require designed experiments on computer models (for example, finite element analysis and computational fluid dynamics), traceability studies to identify the source of a performance problem, or tolerance stack-up analyses. Also, product reliability assessments are usually important for such products. In the processing industries, a frequent statistical challenge is determining and maintaining a mix of product ingredients that optimizes product performance.
Process improvement: Statistics are used extensively to improve business processes. Such projects are often undertaken as Six Sigma projects (which we address in Chapter 9). Some examples include redesigning a billing process to eliminate errors and collect revenues faster, increasing a call center's timeliness in successfully addressing customer issues, and reducing cycle time and shipment errors in filling customer orders.
Field support: The major goal of statistics in field support is to help develop optimum servicing and failure avoidance strategies and to leverage the information to build improved future product by:
• Developing programs to track product field reliability and to identify failures and their causes, address them, and avoid future instances.
• Comparing field performance of a product with competitive products and making statistically valid advertising claims based on the results.
• Establishing proactive product servicing initiatives, such as preventive maintenance and parts replacement, to avoid field failures and to mitigate the impact of failures that do occur.
Many new industries-such as bioinformatics, medical imaging, and nanotechnology-are emerging as part of a long list of industries that employ statistics. Here are a few stand-outs:
• Pharmaceuticals: To ensure that newly introduced pharmaceutical products are proven safe and effective, preclinical and clinical trials need to be designed, executed, and reported. This requires the design and analysis of carefully controlled, statistically planned investigations. Other important statistical applications are in product safety evaluations, manufacturing process improvement, and tracking of long-term field performance.
• Semiconductors: The semiconductor business is characterized by its many process steps and efforts to improve yield and is highly data-intensive. For example, statistics are used to study and understand the impact of process variables at one stage of production on performance at subsequent stages, monitor and control critical process variables, and weed out potential early field failures through the use of product burn-in.
• Communications: Typical applications in this data-intensive industry include the identification of peak usage times and evaluation of capabilities to provide normal service during such times, assessments of software reliability, evaluation of the impact of alternative pricing strategies, and studies of Internet traffic patterns.
• Service industries: There has been growing recognition that statistical concepts and methods can also make important contributions to the operation and improvement of service businesses. The large amount of money at stake in financial transactions has made finance an especially attractive area for statistical modeling. Typical applications include the development of credit scores to determine which applicants should be approved, fraud detection schemes that maximize the identification of culprits while minimizing the number of falsely suspected innocents, marketing strategies that identify existing customers who are likely to be interested in other company products, and assessments of risk.
Why should statistics be taught to, and understood by, employees in all functions and all levels of an organization?
Product design and reliability: The major goal of statistics in product design is to help develop the best possible product and to guide its successful transition to manufacturing. This includes involvement in setting design goals, ensuring measurement capability, designing a product that meets or exceeds the goals, validating the design, and transitioning it to manufacturing. For example, statistics can help:
• Determine customer needs and wishes through market-research studies.
• Evaluate the impact of critical variables on product characteristics and assess robustness to variability in manufacturing conditions and product use through designed experiments.
• Assess and improve product reliability through the design and analysis of accelerated test programs. In the past, reliability activities were mainly reactive, dealing principally with responding to unexpected field failures-for example, quantifying the severity of a problem, assessing whether a recall is needed, and, if so, determining what segment of the product population needs to be recalled. Such problems, unfortunately, still arise today. But the main focus is on avoiding them and learning how to build a consistently high-reli-ability product in the first place.
Many of these concepts are discussed further in Chapter 7.
Manufacturing: The major goal of statistics during manufacturing is to help ensure the building of a consistently high-quality product. Typical applications are to help plan and analyze the results of programs to:
• Assess manufacturing capability and stability.
• Evaluate and remove measurement bias and quantify and reduce measurement variability through gauge repeatability and reproducibility studies.
• Remove deviations from target performance and reduce performance variability.
• Monitor the process-often using control charts -to signal significant changes, to identify and remove special causes of variation, and to provide the path to permanent improvement.
These are discussed further in Chapter 8.
The manufacture of discrete parts often calls for the use of different statistical tools from those used in the processing industries. It may require designed experiments on computer models (for example, finite element analysis and computational fluid dynamics), traceability studies to identify the source of a performance problem, or tolerance stack-up analyses. Also, product reliability assessments are usually important for such products. In the processing industries, a frequent statistical challenge is determining and maintaining a mix of product ingredients that optimizes product performance.
Process improvement: Statistics are used extensively to improve business processes. Such projects are often undertaken as Six Sigma projects (which we address in Chapter 9). Some examples include redesigning a billing process to eliminate errors and collect revenues faster, increasing a call center's timeliness in successfully addressing customer issues, and reducing cycle time and shipment errors in filling customer orders.
Field support: The major goal of statistics in field support is to help develop optimum servicing and failure avoidance strategies and to leverage the information to build improved future product by:
• Developing programs to track product field reliability and to identify failures and their causes, address them, and avoid future instances.
• Comparing field performance of a product with competitive products and making statistically valid advertising claims based on the results.
• Establishing proactive product servicing initiatives, such as preventive maintenance and parts replacement, to avoid field failures and to mitigate the impact of failures that do occur.
Many new industries-such as bioinformatics, medical imaging, and nanotechnology-are emerging as part of a long list of industries that employ statistics. Here are a few stand-outs:
• Pharmaceuticals: To ensure that newly introduced pharmaceutical products are proven safe and effective, preclinical and clinical trials need to be designed, executed, and reported. This requires the design and analysis of carefully controlled, statistically planned investigations. Other important statistical applications are in product safety evaluations, manufacturing process improvement, and tracking of long-term field performance.
• Semiconductors: The semiconductor business is characterized by its many process steps and efforts to improve yield and is highly data-intensive. For example, statistics are used to study and understand the impact of process variables at one stage of production on performance at subsequent stages, monitor and control critical process variables, and weed out potential early field failures through the use of product burn-in.
• Communications: Typical applications in this data-intensive industry include the identification of peak usage times and evaluation of capabilities to provide normal service during such times, assessments of software reliability, evaluation of the impact of alternative pricing strategies, and studies of Internet traffic patterns.
• Service industries: There has been growing recognition that statistical concepts and methods can also make important contributions to the operation and improvement of service businesses. The large amount of money at stake in financial transactions has made finance an especially attractive area for statistical modeling. Typical applications include the development of credit scores to determine which applicants should be approved, fraud detection schemes that maximize the identification of culprits while minimizing the number of falsely suspected innocents, marketing strategies that identify existing customers who are likely to be interested in other company products, and assessments of risk.
Why should statistics be taught to, and understood by, employees in all functions and all levels of an organization?
Explanation
Statistics refers to that form of mathe...
Managing for Quality and Performance Excellence 10th Edition by James Evans ,William Lindsay
Why don’t you like this exercise?
Other Minimum 8 character and maximum 255 character
Character 255