Exam 18: Professional Data Engineer on Google Cloud Platform
Exam 1: Google AdWords: Display Advertising122 Questions
Exam 2: Google AdWords Fundamentals153 Questions
Exam 3: Associate Android Developer86 Questions
Exam 4: Associate Cloud Engineer134 Questions
Exam 5: Cloud Digital Leader91 Questions
Exam 6: Google Analytics Individual Qualification (IQ)121 Questions
Exam 7: Google Analytics Individual Qualification78 Questions
Exam 8: GSuite202 Questions
Exam 9: Looker Business Analyst388 Questions
Exam 10: LookML Developer41 Questions
Exam 11: Mobile Web Specialist13 Questions
Exam 12: Professional Cloud Architect on Google Cloud Platform118 Questions
Exam 13: Professional Cloud Developer85 Questions
Exam 14: Professional Cloud DevOps Engineer28 Questions
Exam 15: Professional Cloud Network Engineer57 Questions
Exam 16: Professional Cloud Security Engineer80 Questions
Exam 17: Professional Collaboration Engineer71 Questions
Exam 18: Professional Data Engineer on Google Cloud Platform256 Questions
Exam 19: Professional Machine Learning Engineer35 Questions
Select questions type
A data scientist has created a BigQuery ML model and asks you to create an ML pipeline to serve predictions. You have a REST API application with the requirement to serve predictions for an individual user ID with latency under 100 milliseconds. You use the following query to generate predictions: SELECT predicted_label, user_id FROM ML.PREDICT (MODEL 'dataset.model', table user_features) . How should you create the ML pipeline?
(Multiple Choice)
4.9/5
(26)
Your neural network model is taking days to train. You want to increase the training speed. What can you do?
(Multiple Choice)
4.9/5
(27)
When you design a Google Cloud Bigtable schema it is recommended that you _________.
(Multiple Choice)
4.8/5
(38)
Which of the following job types are supported by Cloud Dataproc (select 3 answers)?
(Multiple Choice)
4.8/5
(36)
You're training a model to predict housing prices based on an available dataset with real estate properties. Your plan is to train a fully connected neural net, and you've discovered that the dataset contains latitude and longitude of the property. Real estate professionals have told you that the location of the property is highly influential on price, so you'd like to engineer a feature that incorporates this physical dependency. What should you do?
(Multiple Choice)
4.7/5
(36)
You work for a large real estate firm and are preparing 6 TB of home sales data to be used for machine learning. You will use SQL to transform the data and use BigQuery ML to create a machine learning model. You plan to use the model for predictions against a raw dataset that has not been transformed. How should you set up your workflow in order to prevent skew at prediction time?
(Multiple Choice)
4.9/5
(35)
A shipping company has live package-tracking data that is sent to an Apache Kafka stream in real time. This is then loaded into BigQuery. Analysts in your company want to query the tracking data in BigQuery to analyze geospatial trends in the lifecycle of a package. The table was originally created with ingest-date partitioning. Over time, the query processing time has increased. You need to implement a change that would improve query performance in BigQuery. What should you do?
(Multiple Choice)
4.8/5
(37)
Does Dataflow process batch data pipelines or streaming data pipelines?
(Multiple Choice)
4.8/5
(37)
You are responsible for writing your company's ETL pipelines to run on an Apache Hadoop cluster. The pipeline will require some checkpointing and splitting pipelines. Which method should you use to write the pipelines?
(Multiple Choice)
5.0/5
(39)
You are creating a model to predict housing prices. Due to budget constraints, you must run it on a single resource-constrained virtual machine. Which learning algorithm should you use?
(Multiple Choice)
4.8/5
(27)
You are deploying a new storage system for your mobile application, which is a media streaming service. You decide the best fit is Google Cloud Datastore. You have entities with multiple properties, some of which can take on multiple values. For example, in the entity 'Movie' the property 'actors' and the property 'tags' have multiple values but the property 'date released' does not. A typical query would ask for all movies with actor=<actorname> ordered by date _ released or all movies with tag=Comedy date_released. How should you avoid a combinatorial explosion in the number of indexes?
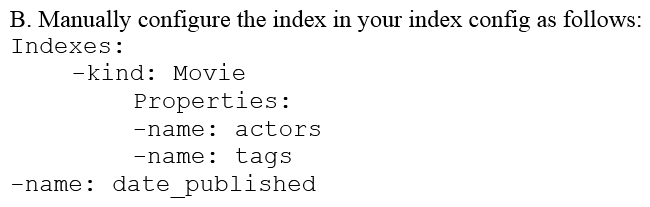
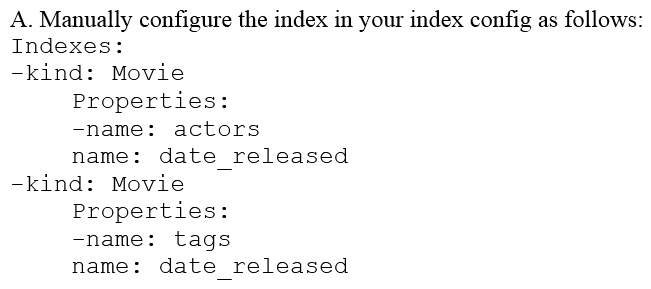
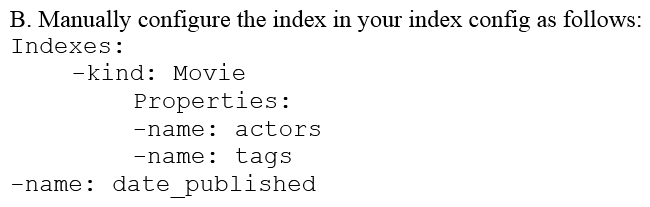
(Multiple Choice)
4.8/5
(32)
For the best possible performance, what is the recommended zone for your Compute Engine instance and Cloud Bigtable instance?
(Multiple Choice)
4.8/5
(34)
As your organization expands its usage of GCP, many teams have started to create their own projects. Projects are further multiplied to accommodate different stages of deployments and target audiences. Each project requires unique access control configurations. The central IT team needs to have access to all projects. Furthermore, data from Cloud Storage buckets and BigQuery datasets must be shared for use in other projects in an ad hoc way. You want to simplify access control management by minimizing the number of policies. Which two steps should you take? Choose 2 answers.
(Multiple Choice)
4.8/5
(35)
You are designing a basket abandonment system for an ecommerce company. The system will send a message to a user based on these rules: No interaction by the user on the site for 1 hour Has added more than $30 worth of products to the basket Has not completed a transaction You use Google Cloud Dataflow to process the data and decide if a message should be sent. How should you design the pipeline?
(Multiple Choice)
4.9/5
(28)
After migrating ETL jobs to run on BigQuery, you need to verify that the output of the migrated jobs is the same as the output of the original. You've loaded a table containing the output of the original job and want to compare the contents with output from the migrated job to show that they are identical. The tables do not contain a primary key column that would enable you to join them together for comparison. What should you do?
(Multiple Choice)
4.8/5
(35)
You are analyzing the price of a company's stock. Every 5 seconds, you need to compute a moving average of the past 30 seconds' worth of data. You are reading data from Pub/Sub and using DataFlow to conduct the analysis. How should you set up your windowed pipeline?
(Multiple Choice)
4.9/5
(38)
You are designing a cloud-native historical data processing system to meet the following conditions: The data being analyzed is in CSV, Avro, and PDF formats and will be accessed by multiple analysis tools including Cloud Dataproc, BigQuery, and Compute Engine. A streaming data pipeline stores new data daily. Peformance is not a factor in the solution. The solution design should maximize availability. How should you design data storage for this solution?
(Multiple Choice)
4.9/5
(34)
You need to create a data pipeline that copies time-series transaction data so that it can be queried from within BigQuery by your data science team for analysis. Every hour, thousands of transactions are updated with a new status. The size of the intitial dataset is 1.5 PB, and it will grow by 3 TB per day. The data is heavily structured, and your data science team will build machine learning models based on this data. You want to maximize performance and usability for your data science team. Which two strategies should you adopt? Choose 2 answers.
(Multiple Choice)
5.0/5
(38)
MJTelco Case Study Company Overview MJTelco is a startup that plans to build networks in rapidly growing, underserved markets around the world. The company has patents for innovative optical communications hardware. Based on these patents, they can create many reliable, high-speed backbone links with inexpensive hardware. Company Background Founded by experienced telecom executives, MJTelco uses technologies originally developed to overcome communications challenges in space. Fundamental to their operation, they need to create a distributed data infrastructure that drives real-time analysis and incorporates machine learning to continuously optimize their topologies. Because their hardware is inexpensive, they plan to overdeploy the network allowing them to account for the impact of dynamic regional politics on location availability and cost. Their management and operations teams are situated all around the globe creating many-to-many relationship between data consumers and provides in their system. After careful consideration, they decided public cloud is the perfect environment to support their needs. Solution Concept MJTelco is running a successful proof-of-concept (PoC) project in its labs. They have two primary needs: Scale and harden their PoC to support significantly more data flows generated when they ramp to more than 50,000 installations. Refine their machine-learning cycles to verify and improve the dynamic models they use to control topology definition. MJTelco will also use three separate operating environments - development/test, staging, and production - to meet the needs of running experiments, deploying new features, and serving production customers. Business Requirements Scale up their production environment with minimal cost, instantiating resources when and where needed in an unpredictable, distributed telecom user community. Ensure security of their proprietary data to protect their leading-edge machine learning and analysis. Provide reliable and timely access to data for analysis from distributed research workers Maintain isolated environments that support rapid iteration of their machine-learning models without affecting their customers. Technical Requirements Ensure secure and efficient transport and storage of telemetry data Rapidly scale instances to support between 10,000 and 100,000 data providers with multiple flows each. Allow analysis and presentation against data tables tracking up to 2 years of data storing approximately 100m records/day Support rapid iteration of monitoring infrastructure focused on awareness of data pipeline problems both in telemetry flows and in production learning cycles. CEO Statement Our business model relies on our patents, analytics and dynamic machine learning. Our inexpensive hardware is organized to be highly reliable, which gives us cost advantages. We need to quickly stabilize our large distributed data pipelines to meet our reliability and capacity commitments. CTO Statement Our public cloud services must operate as advertised. We need resources that scale and keep our data secure. We also need environments in which our data scientists can carefully study and quickly adapt our models. Because we rely on automation to process our data, we also need our development and test environments to work as we iterate. CFO Statement The project is too large for us to maintain the hardware and software required for the data and analysis. Also, we cannot afford to staff an operations team to monitor so many data feeds, so we will rely on automation and infrastructure. Google Cloud's machine learning will allow our quantitative researchers to work on our high-value problems instead of problems with our data pipelines. You need to compose visualizations for operations teams with the following requirements: The report must include telemetry data from all 50,000 installations for the most resent 6 weeks (sampling once every minute). The report must not be more than 3 hours delayed from live data. The actionable report should only show suboptimal links. Most suboptimal links should be sorted to the top. Suboptimal links can be grouped and filtered by regional geography. User response time to load the report must be <5 seconds. Which approach meets the requirements?
(Multiple Choice)
4.8/5
(32)
Showing 201 - 220 of 256
Filters
- Essay(0)
- Multiple Choice(0)
- Short Answer(0)
- True False(0)
- Matching(0)