Exam 14: Introduction to Multiple Regression
Exam 1: Instruction and Data Collection47 Questions
Exam 2: Presenting Data in Tables and Charts277 Questions
Exam 3: Numerical Descriptive Measures139 Questions
Exam 4: Basic Probability137 Questions
Exam 5: Some Important Discrete Probability Distributions188 Questions
Exam 6: The Normal Distribution and Other Continuous Distributions164 Questions
Exam 7: Sampling and Sampling Distributions187 Questions
Exam 8: Confidence Interval Estimation173 Questions
Exam 9: Fundamentals of Hypothesis Testing: One-Sample Tests146 Questions
Exam 10: Two-Sample Tests190 Questions
Exam 11: Analysis of Variance127 Questions
Exam 12: Chi-Square Tests and Nonparametric Tests174 Questions
Exam 13: Simple Linear Regression198 Questions
Exam 14: Introduction to Multiple Regression215 Questions
Exam 15: Multiple Regression Model Building101 Questions
Exam 16: Time-Series Analysis and Index Numbers133 Questions
Exam 17: Statistical Applications in Quality Management132 Questions
Exam 18: Data Analysis Overview52 Questions
Select questions type
TABLE 14-10
You worked as an intern at We Always Win Car Insurance Company last summer. You notice that individual car insurance premiums depend very much on the age of the individual, the number of traffic tickets received by the individual, and the population density of the city in which the individual lives. You performed a regression analysis in EXCEL and obtained the following information:
-Referring to Table 14-10, to test the significance of the multiple regression model, the p-value of the test statistic in the sample is ________.
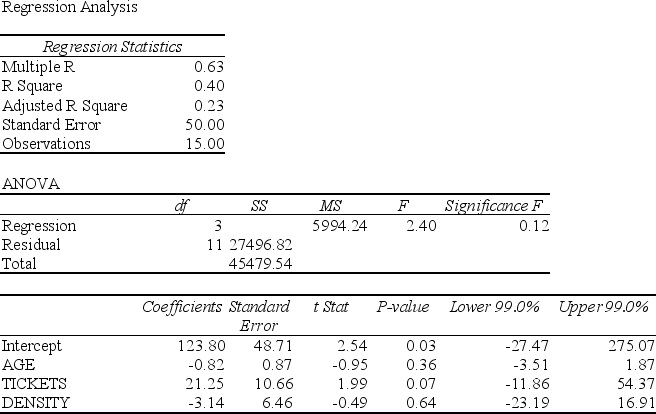
(Short Answer)
4.9/5
(34)
TABLE 14-15
The superintendent of a school district wanted to predict the percentage of students passing a sixth-grade proficiency test. She obtained the data on percentage of students passing the proficiency test (% Passing), daily average of the percentage of students attending class (% Attendance), average teacher salary in dollars (Salaries), and instructional spending per pupil in dollars (Spending) of 47 schools in the state.
Following is the multiple regression output with Y = % Passing as the dependent variable, X1= % Attendance, X2= Salaries and X3= Spending:
Note:
-Referring to Table 14-15, the null hypothesis H0 : β1 = β2 = β3 = 0 implies that percentage of students passing the proficiency test is not affected by any of the explanatory variables.
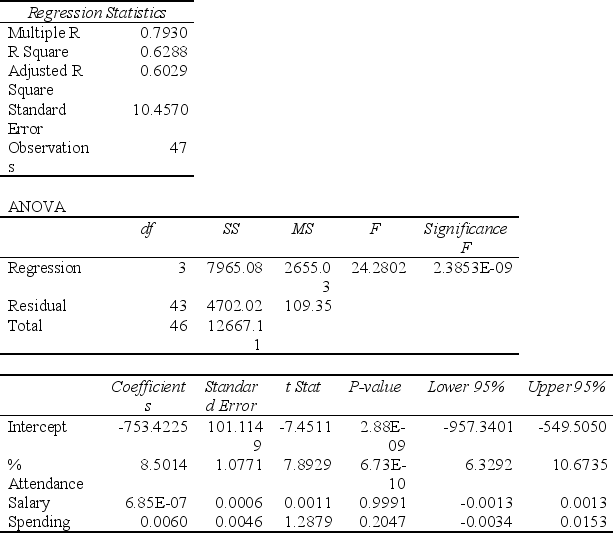

(True/False)
4.8/5
(30)
TABLE 14-15
The superintendent of a school district wanted to predict the percentage of students passing a sixth-grade proficiency test. She obtained the data on percentage of students passing the proficiency test (% Passing), daily average of the percentage of students attending class (% Attendance), average teacher salary in dollars (Salaries), and instructional spending per pupil in dollars (Spending) of 47 schools in the state.
Following is the multiple regression output with Y = % Passing as the dependent variable, X1= % Attendance, X2= Salaries and X3= Spending:
Note:
-Referring to Table 14-15, we can conclude that instructional spending per pupil has no impact on the mean percentage of students passing the proficiency test at a 5% level of significance using the 95% confidence interval estimate for β3.
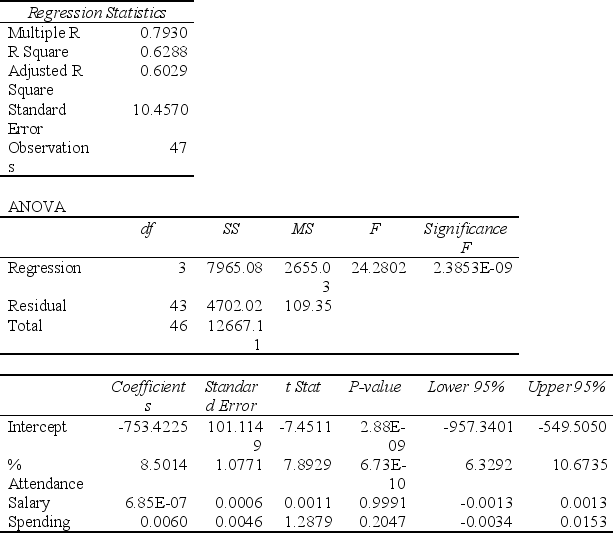

(True/False)
4.8/5
(33)
TABLE 14-8
A financial analyst wanted to examine the relationship between salary (in $1,000) and 4 variables: age (X1 = Age), experience in the field (X2 = Exper), number of degrees (X3 = Degrees), and number of previous jobs in the field (X4 = Prevjobs). He took a sample of 20 employees and obtained the following Microsoft Excel output:
SUMMARY OUTPUT
Regression Statistics
ANOVA
-Referring to Table 14-8, the analyst decided to obtain a 99% confidence interval for β3. The confidence interval is from ________ to ________.
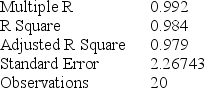

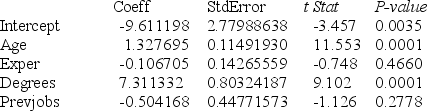
(Short Answer)
4.8/5
(36)
A regression had the following results: SST = 82.55, SSE = 29.85. It can be said that 63.84% of the variation in the dependent variable is explained by the independent variables in the regression.
(True/False)
4.7/5
(28)
TABLE 14-4
A real estate builder wishes to determine how house size (House) is influenced by family income (Income), family size (Size), and education of the head of household (School). House size is measured in hundreds of square feet, income is measured in thousands of dollars, and education is in years. The builder randomly selected 50 families and ran the multiple regression. Microsoft Excel output is provided below:
SUMMARY OUTPUT
Regression Statistics
ANOVA
-Referring to Table 14-4, one individual in the sample had an annual income of $10,000, a family size of 1, and an education of 8 years. This individual owned a home with an area of 1,000 square feet (House = 10.00). What is the residual (in hundreds of square feet) for this data point?
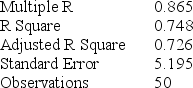


(Multiple Choice)
4.8/5
(40)
TABLE 14-15
The superintendent of a school district wanted to predict the percentage of students passing a sixth-grade proficiency test. She obtained the data on percentage of students passing the proficiency test (% Passing), daily average of the percentage of students attending class (% Attendance), average teacher salary in dollars (Salaries), and instructional spending per pupil in dollars (Spending) of 47 schools in the state.
Following is the multiple regression output with Y = % Passing as the dependent variable, X1= % Attendance, X2= Salaries and X3= Spending:
Note:
-Referring to Table 14-15, what is the value of the test statistics when testing whether instructional spending per pupil has any effect on percentage of students passing the proficiency test?
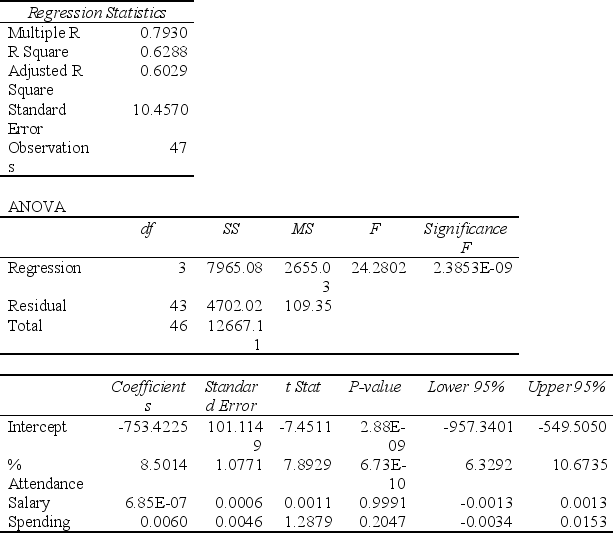

(Short Answer)
4.7/5
(43)
TABLE 14-3
An economist is interested to see how consumption for an economy (in $ billions) is influenced by gross domestic product ($ billions) and aggregate price (consumer price index). The Microsoft Excel output of this regression is partially reproduced below.
SUMMARY OUTPUT
Regression Statistics
ANOVA
-Referring to Table 14-3, what is the estimated mean consumption level for an economy with GDP equal to $2 billion and an aggregate price index of 90?
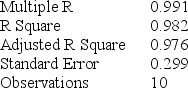


(Multiple Choice)
4.7/5
(37)
The slopes in a multiple regression model are called net regression coefficients.
(True/False)
4.8/5
(40)
When an additional explanatory variable is introduced into a multiple regression model, the coefficient of multiple determination will never decrease.
(True/False)
4.9/5
(27)
TABLE 14-13
An econometrician is interested in evaluating the relation of demand for building materials to mortgage rates in Los Angeles and San Francisco. He believes that the appropriate model is
-Referring to Table 14-13, the effect of living in San Francisco rather than Los Angeles is to increase the mean demand by an estimated ________.


(Short Answer)
4.8/5
(25)
TABLE 14-15
The superintendent of a school district wanted to predict the percentage of students passing a sixth-grade proficiency test. She obtained the data on percentage of students passing the proficiency test (% Passing), daily average of the percentage of students attending class (% Attendance), average teacher salary in dollars (Salaries), and instructional spending per pupil in dollars (Spending) of 47 schools in the state.
Following is the multiple regression output with Y = % Passing as the dependent variable, X1= % Attendance, X2= Salaries and X3= Spending:
Note:
-Referring to Table 14-15, the null hypothesis should be rejected at a 5% level of significance when testing whether there is a significant relationship between percentage of students passing the proficiency test and the entire set of explanatory variables.
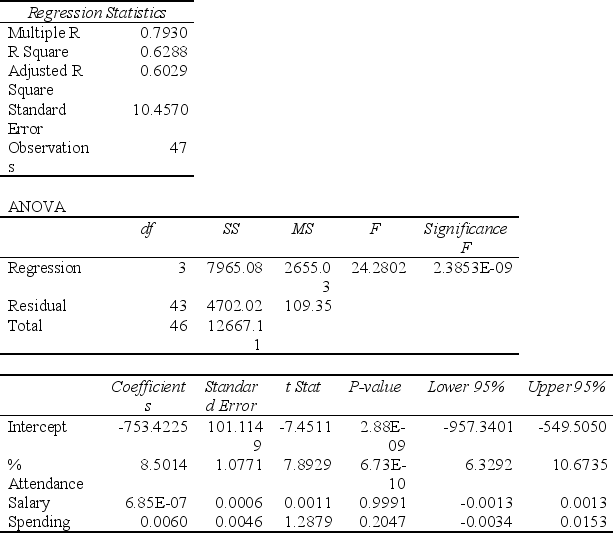

(True/False)
4.7/5
(43)
TABLE 14-5
A microeconomist wants to determine how corporate sales are influenced by capital and wage spending by companies. She proceeds to randomly select 26 large corporations and record information in millions of dollars. The Microsoft Excel output below shows results of this multiple regression.
SUMMARY OUTPUT
Regression Statistics
ANOVA
-Referring to Table 14-5, when the microeconomist used a simple linear regression model with sales as the dependent variable and wages as the independent variable, she obtained an r2 value of 0.601. What additional percentage of the total variation of sales has been explained by including capital spending in the multiple regression?
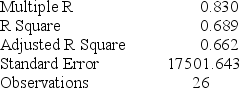


(Multiple Choice)
4.7/5
(30)
TABLE 14-15
The superintendent of a school district wanted to predict the percentage of students passing a sixth-grade proficiency test. She obtained the data on percentage of students passing the proficiency test (% Passing), daily average of the percentage of students attending class (% Attendance), average teacher salary in dollars (Salaries), and instructional spending per pupil in dollars (Spending) of 47 schools in the state.
Following is the multiple regression output with Y = % Passing as the dependent variable, X1= % Attendance, X2= Salaries and X3= Spending:
Note:
-Referring to Table 14-15, we can conclude that average teacher salary has no impact on the mean percentage of students passing the proficiency test at a 1% level of significance based solely on the 95% confidence interval estimate for β2.
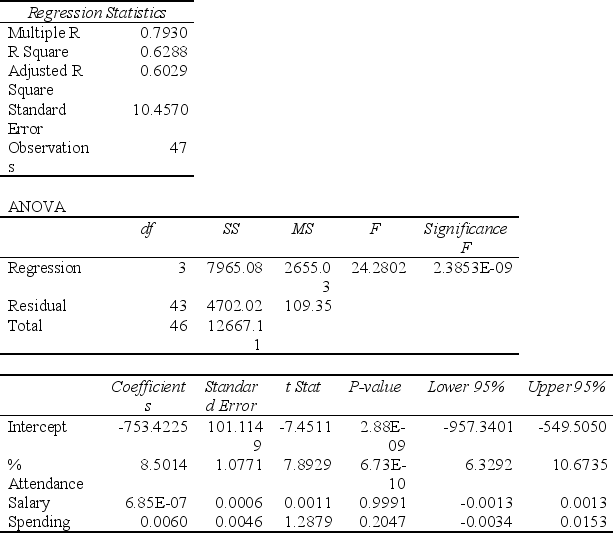

(True/False)
4.8/5
(37)
TABLE 14-11
A weight-loss clinic wants to use regression analysis to build a model for weight-loss of a client (measured in pounds). Two variables thought to affect weight loss are client's length of time on the weight loss program and time of session. These variables are described below:
Data for 12 clients on a weight-loss program at the clinic were collected and used to fit the interaction model:
Partial output from Microsoft Excel follows:
Regression Statistics
ANOVA
-Referring to Table 14-11, in terms of the β's in the model, give the average change in weight-loss (Y) for every 1 month increase in time in the program (X1) when attending the evening session.


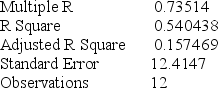
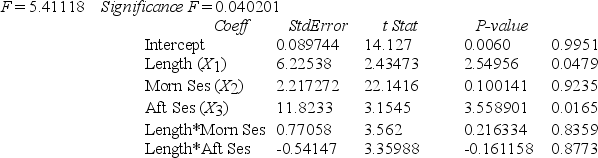
(Multiple Choice)
4.9/5
(32)
TABLE 14-3
An economist is interested to see how consumption for an economy (in $ billions) is influenced by gross domestic product ($ billions) and aggregate price (consumer price index). The Microsoft Excel output of this regression is partially reproduced below.
SUMMARY OUTPUT
Regression Statistics
ANOVA
-Referring to Table 14-3, one economy in the sample had an aggregate consumption level of $3 billion, a GDP of $3.5 billion, and an aggregate price level of 125. What is the residual for this data point?
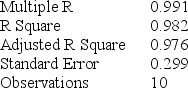


(Multiple Choice)
4.9/5
(39)
TABLE 14-4
A real estate builder wishes to determine how house size (House) is influenced by family income (Income), family size (Size), and education of the head of household (School). House size is measured in hundreds of square feet, income is measured in thousands of dollars, and education is in years. The builder randomly selected 50 families and ran the multiple regression. Microsoft Excel output is provided below:
SUMMARY OUTPUT
Regression Statistics
ANOVA
-Referring to Table 14-4, what is the predicted house size (in hundreds of square feet) for an individual earning an annual income of $40,000, having a family size of 4, and going to school a total of 13 years?
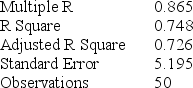


(Multiple Choice)
4.7/5
(32)
Consider a regression in which b2 = -1.5 and the standard error of this coefficient equals 0.3. To determine whether X2 is a significant explanatory variable, you would compute an observed t-value of -5.0.
(True/False)
4.8/5
(30)
An interaction term in a multiple regression model may be used when the relationship between X1 and Y changes for differing values of X2.
(True/False)
4.8/5
(32)
TABLE 14-11
A weight-loss clinic wants to use regression analysis to build a model for weight-loss of a client (measured in pounds). Two variables thought to affect weight loss are client's length of time on the weight loss program and time of session. These variables are described below:
Data for 12 clients on a weight-loss program at the clinic were collected and used to fit the interaction model:
Partial output from Microsoft Excel follows:
Regression Statistics
ANOVA
-In a multiple regression model, the adjusted r2


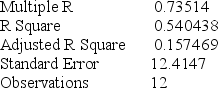
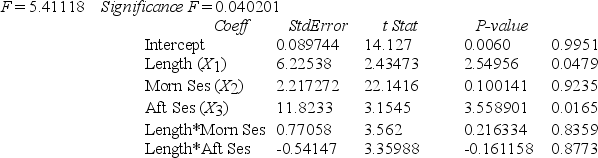
(Multiple Choice)
4.8/5
(33)
Showing 161 - 180 of 215
Filters
- Essay(0)
- Multiple Choice(0)
- Short Answer(0)
- True False(0)
- Matching(0)