Exam 8: Nonlinear Regression Functions
Exam 1: Economic Questions and Data17 Questions
Exam 2: Review of Probability70 Questions
Exam 3: Review of Statistics65 Questions
Exam 4: Linear Regression With One Regressor65 Questions
Exam 5: Regression With a Single Regressor: Hypothesis Tests and Confidence Intervals59 Questions
Exam 6: Linear Regression With Multiple Regressors65 Questions
Exam 7: Hypothesis Tests and Confidence Intervals in Multiple Regression64 Questions
Exam 8: Nonlinear Regression Functions63 Questions
Exam 9: Assessing Studies Based on Multiple Regression65 Questions
Exam 10: Regression With Panel Data50 Questions
Exam 11: Regression With a Binary Dependent Variable50 Questions
Exam 12: Instrumental Variables Regression50 Questions
Exam 13: Experiments and Quasi-Experiments50 Questions
Exam 14: Introduction to Time Series Regression and Forecasting50 Questions
Exam 15: Estimation of Dynamic Causal Effects50 Questions
Exam 16: Additional Topics in Time Series Regression50 Questions
Exam 17: The Theory of Linear Regression With One Regressor49 Questions
Exam 18: The Theory of Multiple Regression50 Questions
Select questions type
To test whether or not the population regression function is linear rather than a polynomial of order r,
(Multiple Choice)
4.9/5
(27)
The figure shows is a plot and a fitted linear regression line of the age-earnings profile of 1,744 individuals, taken from the Current Population Survey.
(a)Describe the problems in predicting earnings using the fitted line. What would the pattern of the residuals look like for the age category under 40?
(b)What alternative functional form might fit the data better?
(c)What other variables might you want to consider in specifying the determinants of earnings?
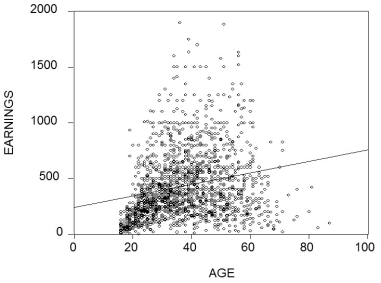
(Essay)
4.9/5
(37)
The interpretation of the slope coefficient in the model Yi = β0 + β1 ln(Xi)+ ui is as follows:
(Multiple Choice)
4.9/5
(32)
In the model Yi = ?0 + ?1X1 + ?2X2 + ?3(X1 × X2)+ ui, the expected effect is
(Multiple Choice)
4.8/5
(37)
The following interactions between binary and continuous variables are possible, with the exception of
(Multiple Choice)
4.9/5
(42)
Table 8.1 on page 284 of your textbook displays the following estimated earnings function in column (4): = 1.503+0.1032\times educ -0.451\times DFemme +0.0143\times( DFemme \times educ ) (0.023)(0.0012)(0.024)(0.0017)
+0.0232\times exper -0.000368\times exper 2-0.058\times Midwest -0.0098\times South -0.030\times West (0.0012)(0.000023)(0.006)(0.006)(0.007)
Given that the potential experience variable (exper)is defined as (Age-Education-6)find the age at which individuals with a high school degree (12 years of education)and with a college degree (16 years of education)have maximum earnings, holding all other factors constant.
(Short Answer)
4.9/5
(31)
(Requires Calculus)In the equation = 607.3 + 3.85 Income - 0.0423Income2, the following income level results in the maximum test score
(Multiple Choice)
4.8/5
(42)
Many countries that experience hyperinflation do not have market-determined interest rates. As a result, some authors have substituted future inflation rates into money demand equations of the following type as a proxy: (m is real money, and P is the consumer price index).
Income is typically omitted since movements in it are dwarfed by money growth and the inflation rate. Authors have then interpreted β1 as the "semi-elasticity" of the inflation rate. Do you see any problems with this interpretation?
(Essay)
4.9/5
(38)
In the regression model Yi = β0 + β1Xi + β2Di + β3(Xi × Di)+ ui, where X is a continuous variable and D is a binary variable, β2
(Multiple Choice)
4.9/5
(36)
To decide whether Yi = β0 + β1X + ui or ln(Yi)= β0 + β1X + ui fits the data better, you cannot consult the regression R2 because
(Multiple Choice)
4.8/5
(36)
In estimating the original relationship between money wage growth and the unemployment rate, Phillips used United Kingdom data from 1861 to 1913 to fit a curve of the following functional form
( + β0)= β1 × × eu,
where is the percentage change in money wages and ur is the unemployment rate. Sketch the function. What role does β0 play? Can you find a linear transformation that allows you to estimate the above function using OLS? If, after taking logarithms on both sides of the equation, you tried to estimate β1 and β2 using OLS by choosing different values for β0 by "trial and error procedure" (Phillips's words), what sort of problem might you run into with the left-hand side variable for some of the observations?
(Essay)
4.9/5
(35)
Give at least three examples from economics where you expect some nonlinearity in the relationship between variables. Interpret the slope in each case.
(Essay)
4.8/5
(40)
One of the most frequently estimated equations in the macroeconomics growth literature are so-called convergence regressions. In essence the average per capita income growth rate is regressed on the beginning-of-period per capita income level to see if countries that were further behind initially, grew faster. Some macroeconomic models make this prediction, once other variables are controlled for. To investigate this matter, you collect data from 104 countries for the sample period 1960-1990 and estimate the following relationship (numbers in parentheses are for heteroskedasticity-robust standard errors): = 0.020-0.360\times gpop +0.004\times Educ -0.053\times,=0.332, SER =0.013 (0.009)(0.241)(0.001)(0.009)
where g6090 is the growth rate of GDP per worker for the 1960-1990 sample period, RelProd60 is the initial starting level of GDP per worker relative to the United States in 1960, gpop is the average population growth rate of the country, and Educ is educational attainment in years for 1985.
(a)What is the effect of an increase of 5 years in educational attainment? What would happen if a country could implement policies to cut population growth by one percent? Are all coefficients significant at the 5% level? If one of the coefficients is not significant, should you automatically eliminate its variable from the list of explanatory variables?
(b)The coefficient on the initial condition has to be significantly negative to suggest conditional convergence. Furthermore, the larger this coefficient, in absolute terms, the faster the convergence will take place. It has been suggested to you to interact education with the initial condition to test for additional effects of education on growth. To test for this possibility, you estimate the following regression: =0.015-0.323\times gpop +0.005\times Educ -0.051\times (0.009)(0.238)(0.001)(0.013) -0.0028\times( EducRelProd 60),=0.346,=0.013 (0.0015)
Write down the effect of an additional year of education on growth. West Germany has a value for RelProd60 of 0.57, while Brazil's value is 0.23. What is the predicted growth rate effect of adding one year of education in both countries? Does this predicted growth rate make sense?
(c)What is the implication for the speed of convergence? Is the interaction effect statistically significant?
(d)Convergence regressions are basically of the type
?ln Yt = ?0 - ?1 ln Y0
where ? might be the change over a longer time period, 30 years, say, and the average growth rate is used on the left-hand side. You note that the equation can be rewritten as
?ln Yt = ?0 - (1 - ?1)ln Y0
Over a century ago, Sir Francis Galton first coined the term "regression" by analyzing the relationship between the height of children and the height of their parents. Estimating a function of the type above, he found a positive intercept and a slope between zero and one. He therefore concluded that heights would revert to the mean. Since ultimately this would imply the height of the population being the same, his result has become known as "Galton's Fallacy." Your estimate of ?1 above is approximately 0.05. Do you see a parallel to Galton's Fallacy?
(Essay)
4.8/5
(33)
Earnings functions attempt to predict the log of earnings from a set of explanatory variables, both binary and continuous. You have allowed for an interaction between two continuous variables: education and tenure with the current employer. Your estimated equation is of the following type: = 0 + 1 × Femme + 2 × Educ + 3 × Tenure + 4 x (Educ × Tenure)+ ∙∙∙
where Femme is a binary variable taking on the value of one for females and is zero otherwise, Educ is the number of years of education, and tenure is continuous years of work with the current employer. What is the effect of an additional year of education on earnings ("returns to education")for men? For women? If you allowed for the returns to education to differ for males and females, how would you respecify the above equation? What is the effect of an additional year of tenure with a current employer on earnings?
(Essay)
4.9/5
(36)
Earnings functions attempt to find the determinants of earnings, using both continuous and binary variables. One of the central questions analyzed in this relationship is the returns to education.
(a)Collecting data from 253 individuals, you estimate the following relationship
=0.54+0.083\timesEduc,=0.20,SER=0.445 (0.14)(0.011)
where Earn is average hourly earnings and Educ is years of education.
What is the effect of an additional year of schooling? If you had a strong belief that years of high school education were different from college education, how would you modify the equation? What if your theory suggested that there was a "diploma effect"?
(b)You read in the literature that there should also be returns to on-the-job training. To approximate on-the-job training, researchers often use the so called Mincer or potential experience variable, which is defined as Exper = Age - Educ - 6. Explain the reasoning behind this approximation. Is it likely to resemble years of employment for various sub-groups of the labor force?
(c)You incorporate the experience variable into your original regression =-0.01+0.101\times Educ +0.033\times Exper -0.0005\times Exper 2
( 0.16 ) ( 0.012 ) (0.006) (0.0001)
What is the effect of an additional year of experience for a person who is 40 years old and had 12 years of education? What about for a person who is 60 years old with the same education background?
(d)Test for the significance of each of the coefficients of the added variables. Why has the coefficient on education changed so little? Sketch the age-(log)earnings profile for workers with 8 years of education and 16 years of education.
(e)You want to find the effect of introducing two variables, gender and marital status. Accordingly you specify a binary variable that takes on the value of one for females and is zero otherwise (Female), and another binary variable that is one if the worker is married but is zero otherwise (Married). Adding these variables to the regressors results in: =0.21+0.093\times Educ +0.032\times Exper -0.0005\times (0.16) (0.012)(0.006)(0.0001) -0.289\times Female +0.062 Married, (0.049) (0.056) =0.43,SER=0.378
Are the coefficients of the two added binary variables individually statistically significant? Are they economically important? In percentage terms, how much less do females earn per hour, controlling for education and experience? How much more do married people make? What is the percentage difference in earnings between a single male and a married female? What is the marriage differential between males and females?
(f)In your final specification, you allow for the binary variables to interact. The results are as follows: =0.14+0.093\times Educ +0.032\times Exper -0.0005\times Exper 2 (0.16) (0.011)(0.006)(0.001) -0.158\times Female +0.173\times Married -0.218\times( Female \times Married ) , (0.075) (0.080)(0.097)
Repeat the exercise in (e)of calculating the various percentage differences between gender and marital status.
(Essay)
4.8/5
(38)
In the case of perfect multicollinearity, OLS is unable to estimate the slope coefficients of the variables involved. Assume that you have included both X1 and X2 as explanatory variables, and that X2 = X 2 1 , so that there is an exact relationship between two explanatory variables. Does this pose a problem for estimation?
(Essay)
4.8/5
(40)
Showing 21 - 40 of 63
Filters
- Essay(0)
- Multiple Choice(0)
- Short Answer(0)
- True False(0)
- Matching(0)