Exam 14: Introduction to Multiple
Exam 1: Defining and Collecting Data202 Questions
Exam 2: Organizing and Visualizing256 Questions
Exam 3: Numerical Descriptive Measures217 Questions
Exam 4: Basic Probability167 Questions
Exam 5: Discrete Probability Distributions165 Questions
Exam 6: The Normal Distribution and Other Continuous Distributions170 Questions
Exam 7: Sampling Distributions165 Questions
Exam 8: Confidence Interval Estimation219 Questions
Exam 9: Fundamentals of Hypothesis Testing: One-Sample Tests194 Questions
Exam 10: Two-Sample Tests240 Questions
Exam 11: Analysis of Variance170 Questions
Exam 12: Chi-Square and Nonparametric188 Questions
Exam 13: Simple Linear Regression243 Questions
Exam 14: Introduction to Multiple394 Questions
Exam 15: Multiple Regression146 Questions
Exam 16: Time-Series Forecasting235 Questions
Exam 17: Getting Ready to Analyze Data386 Questions
Exam 18: Statistical Applications in Quality Management159 Questions
Exam 19: Decision Making126 Questions
Exam 20: Probability and Combinatorics421 Questions
Select questions type
SCENARIO 14-15
The superintendent of a school district wanted to predict the percentage of students passing a sixth-
grade proficiency test. She obtained the data on percentage of students passing the proficiency test
(% Passing), mean teacher salary in thousands of dollars (Salaries), and instructional spending per
pupil in thousands of dollars (Spending) of 47 schools in the state. Following is the multiple regression output with Passing as the dependent variable,
Salaries and Spending:
Regression Statistics Multiple R 0.4276 R Square 0.1828 Adjusted R Square 0.1457 Standard Error 5.7351 Observations 47
ANOVA
Coefficients Standard Error t Stat \rho -value Lower 95\% Upper 95\% Intercept -72.9916 45.9106 -1.5899 0.1190 -165.5184 19.5352 Salary 2.7939 0.8974 3.1133 0.0032 0.9853 4.6025 Spending 0.3742 0.9782 0.3825 0.7039 -1.5972 2.3455
-Referring to Scenario 14-15, what are the numerator and denominator degrees of freedom,
respectively, for the test statistic to determine whether there is a significant relationship between
percentage of students passing the proficiency test and the entire set of explanatory variables?

(Short Answer)
4.9/5
(31)
SCENARIO 14-15
The superintendent of a school district wanted to predict the percentage of students passing a sixth-
grade proficiency test. She obtained the data on percentage of students passing the proficiency test
(% Passing), mean teacher salary in thousands of dollars (Salaries), and instructional spending per
pupil in thousands of dollars (Spending) of 47 schools in the state. Following is the multiple regression output with Passing as the dependent variable,
Salaries and Spending:
Regression Statistics Multiple R 0.4276 R Square 0.1828 Adjusted R Square 0.1457 Standard Error 5.7351 Observations 47
ANOVA
Coefficients Standard Error t Stat \rho -value Lower 95\% Upper 95\% Intercept -72.9916 45.9106 -1.5899 0.1190 -165.5184 19.5352 Salary 2.7939 0.8974 3.1133 0.0032 0.9853 4.6025 Spending 0.3742 0.9782 0.3825 0.7039 -1.5972 2.3455
-Referring to Scenario 14-15, there is sufficient evidence that the percentage of
students passing the proficiency test depends on at least one of the explanatory variables at a 5%
level of significance.

(True/False)
4.8/5
(27)
SCENARIO 14-8 A financial analyst wanted to examine the relationship between salary (in ) and 2 variables: age and experience in the field Exper). He took a sample of 20 employees and obtained the following Microsoft Excel output:
Regression Statistics Multiple R 0.8535 R Square 0.7284 Adjusted R Square 0.6964 Standard Error 10.5630 Observations 20
Coefficients Standard Error t Stat P-value Lower 95\% O5\% Intercept 1.5740 9.2723 0.1698 0.8672 -17.9888 21.1368 Age 1.3045 0.1956 6.6678 0.0000 0.8917 1.7173 Exper -0.1478 0.1944 -0.7604 0.4574 -0.5580 0.2624
Also the sum of squares due to the regression for the model that includes only Age is 5022.0654 while the
sum of squares due to the regression for the model that includes only Exper is 125.9848.
-Referring to Scenario 14-8, the analyst wants to use an F-test to test . The
appropriate alternative hypothesis is ________.

(Essay)
4.8/5
(36)
SCENARIO 14-17
Given below are results from the regression analysis where the dependent variable is the number of
weeks a worker is unemployed due to a layoff (Unemploy) and the independent variables are the age
of the worker (Age) and a dummy variable for management position (Manager: 1 = yes, 0 = no).
The results of the regression analysis are given below: \ Regression Statistics Multiple R 0.6391 R Square 0.4085 Adjusted R Square 0.3765 Standard Error 18.8929 Observations 40
Coefficients Standard Error t Stat P-value Intercept -0.2143 11.5796 -0.0185 0.9853 Age 1.4448 0.3160 4.5717 0.0000 Manager -22.5761 11.3488 -1.9893 0.0541
-Referring to Scenario 14-17, what are the lower and upper limits of the 95% confidence
interval estimate for the difference in the mean number of weeks a worker is unemployed due to a
layoff between a worker who is in a management position and one who is not after taking into
consideration the effect of all the other independent variables?

(Short Answer)
4.8/5
(34)
SCENARIO 14-16
What are the factors that determine the acceleration time (in sec.) from 0 to 60 miles per hour of a
car? Data on the following variables for 30 different vehicle models were collected: (Accel Time): Acceleration time in sec.
(Engine Size): c.c.
(Sedan): 1 if the vehicle model is a sedan and 0 otherwise
The regression results using acceleration time as the dependent variable and the remaining variables as the independent variables are presented below.
Regression Statistics Multiple R 0.6096 R Square 0.3716 Adjusted R Square 0.3251 Standard Error 1.4629 Observations 30
ANOVA
Coefficients Standard Error t Stat P-value Lower 95\% Upper 95\% Intercept 7.1052 0.6574 10.8086 0.0000 5.7564 8.4540 Engine Size -0.0005 0.0001 -3.6477 0.0011 -0.0008 -0.0002 Sedan 0.7264 0.5564 1.3056 0.2027 -0.4152 1.8681
-Referring to Scenario 14-16, which of the following assumptions is most likely violated based on the residual plot of the residuals versus predicted Y?

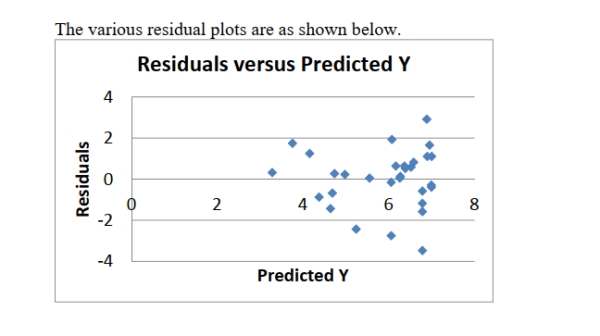
(Multiple Choice)
5.0/5
(34)
SCENARIO 14-20-A
You are the CEO of a dairy company. You are planning to expand milk production by purchasing
additional cows, lands and hiring more workers. From the existing 50 farms owned by the company,
you have collected data on total milk production (in liters), the number of milking cows, land size (in
acres) and the number of laborers. The data are shown below and also available in the Excel file
Scenario14-20-DataA.XLSX.
S
You believe that the number of milking cows , land size and the number of laborers are the best predictors for total milk production on any given farm.
-Referring to Scenario 14-20-A, there is sufficient evidence that total milk
production depends on at least one of the explanatory variables at a 1% level of significance
when testing whether there is a significant relationship between total milk production and the
entire set of explanatory variables.
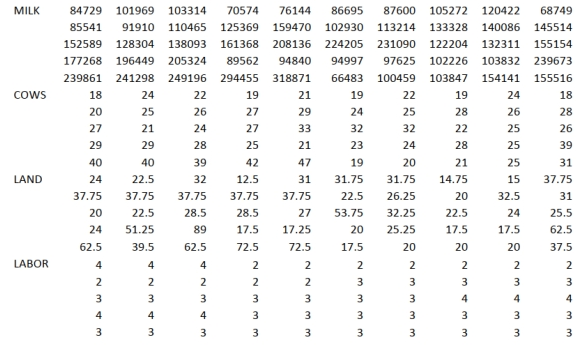
(True/False)
4.9/5
(31)
SCENARIO 14-15
The superintendent of a school district wanted to predict the percentage of students passing a sixth-
grade proficiency test. She obtained the data on percentage of students passing the proficiency test
(% Passing), mean teacher salary in thousands of dollars (Salaries), and instructional spending per
pupil in thousands of dollars (Spending) of 47 schools in the state. Following is the multiple regression output with Passing as the dependent variable,
Salaries and Spending:
Regression Statistics Multiple R 0.4276 R Square 0.1828 Adjusted R Square 0.1457 Standard Error 5.7351 Observations 47
ANOVA
Coefficients Standard Error t Stat \rho -value Lower 95\% Upper 95\% Intercept -72.9916 45.9106 -1.5899 0.1190 -165.5184 19.5352 Salary 2.7939 0.8974 3.1133 0.0032 0.9853 4.6025 Spending 0.3742 0.9782 0.3825 0.7039 -1.5972 2.3455
-Referring to Scenario 14-15, you can conclude that mean
teacher salary has no impact on the mean percentage of students passing the proficiency test,
taking into account the effect of instructional spending per pupil, at a 5% level of significance
using the confidence interval estimate for .

(True/False)
4.8/5
(27)
SCENARIO 14-15
The superintendent of a school district wanted to predict the percentage of students passing a sixth-
grade proficiency test. She obtained the data on percentage of students passing the proficiency test
(% Passing), mean teacher salary in thousands of dollars (Salaries), and instructional spending per
pupil in thousands of dollars (Spending) of 47 schools in the state. Following is the multiple regression output with Passing as the dependent variable,
Salaries and Spending:
Regression Statistics Multiple R 0.4276 R Square 0.1828 Adjusted R Square 0.1457 Standard Error 5.7351 Observations 47
ANOVA
Coefficients Standard Error t Stat \rho -value Lower 95\% Upper 95\% Intercept -72.9916 45.9106 -1.5899 0.1190 -165.5184 19.5352 Salary 2.7939 0.8974 3.1133 0.0032 0.9853 4.6025 Spending 0.3742 0.9782 0.3825 0.7039 -1.5972 2.3455
-Referring to Scenario 14-15, there is sufficient evidence that mean teacher salary
has an effect on percentage of students passing the proficiency test while holding constant the
effect of instructional spending per pupil at a 5% level of significance.

(True/False)
4.8/5
(42)
SCENARIO 14-4
A real estate builder wishes to determine how house size (House) is influenced by family income
(Income) and family size (Size). House size is measured in hundreds of square feet and income is
measured in thousands of dollars. The builder randomly selected 50 families and ran the multiple
regression. Partial Microsoft Excel output is provided below:
-Referring to Scenario 14-4, what are the residual degrees of freedom that are missing from the output?
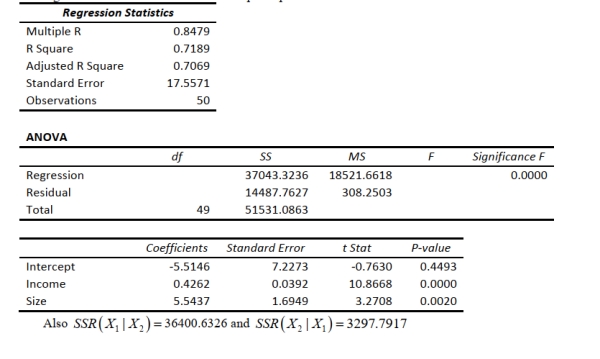
(Multiple Choice)
4.9/5
(26)
SCENARIO 14-16
What are the factors that determine the acceleration time (in sec.) from 0 to 60 miles per hour of a
car? Data on the following variables for 30 different vehicle models were collected: (Accel Time): Acceleration time in sec.
(Engine Size): c.c.
(Sedan): 1 if the vehicle model is a sedan and 0 otherwise
The regression results using acceleration time as the dependent variable and the remaining variables as the independent variables are presented below.
Regression Statistics Multiple R 0.6096 R Square 0.3716 Adjusted R Square 0.3251 Standard Error 1.4629 Observations 30
ANOVA
Coefficients Standard Error t Stat P-value Lower 95\% Upper 95\% Intercept 7.1052 0.6574 10.8086 0.0000 5.7564 8.4540 Engine Size -0.0005 0.0001 -3.6477 0.0011 -0.0008 -0.0002 Sedan 0.7264 0.5564 1.3056 0.2027 -0.4152 1.8681
-Referring to Scenario 14-16, ________ of the variation in Accel Time can be explained by
engine size while controlling for the other independent variable.

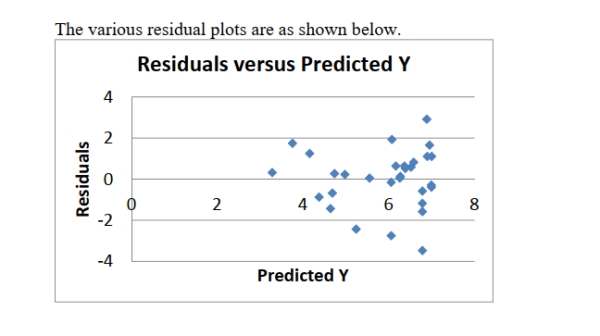
(Short Answer)
4.8/5
(40)
SCENARIO 14-16
What are the factors that determine the acceleration time (in sec.) from 0 to 60 miles per hour of a
car? Data on the following variables for 30 different vehicle models were collected: (Accel Time): Acceleration time in sec.
(Engine Size): c.c.
(Sedan): 1 if the vehicle model is a sedan and 0 otherwise
The regression results using acceleration time as the dependent variable and the remaining variables as the independent variables are presented below.
Regression Statistics Multiple R 0.6096 R Square 0.3716 Adjusted R Square 0.3251 Standard Error 1.4629 Observations 30
ANOVA
Coefficients Standard Error t Stat P-value Lower 95\% Upper 95\% Intercept 7.1052 0.6574 10.8086 0.0000 5.7564 8.4540 Engine Size -0.0005 0.0001 -3.6477 0.0011 -0.0008 -0.0002 Sedan 0.7264 0.5564 1.3056 0.2027 -0.4152 1.8681
-Referring to Scenario 14-16, ________ of the variation in Accel Time can be explained by the
two independent variables.

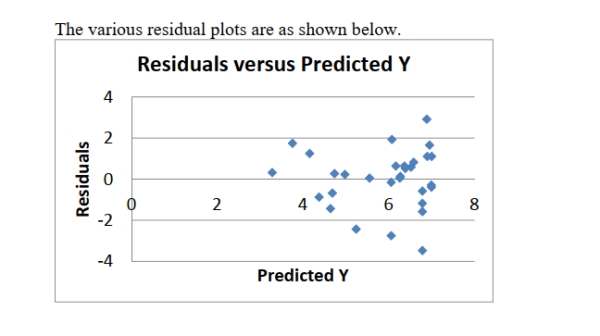
(Short Answer)
4.8/5
(40)
SCENARIO 14-17
Given below are results from the regression analysis where the dependent variable is the number of
weeks a worker is unemployed due to a layoff (Unemploy) and the independent variables are the age
of the worker (Age) and a dummy variable for management position (Manager: 1 = yes, 0 = no).
The results of the regression analysis are given below: \ Regression Statistics Multiple R 0.6391 R Square 0.4085 Adjusted R Square 0.3765 Standard Error 18.8929 Observations 40
Coefficients Standard Error t Stat P-value Intercept -0.2143 11.5796 -0.0185 0.9853 Age 1.4448 0.3160 4.5717 0.0000 Manager -22.5761 11.3488 -1.9893 0.0541
-Referring to Scenario 14-17, which of the following is the correct null hypothesis to determine whether there is a significant relationship between the number of weeks a worker is unemployed
Due to a layoff and the entire set of explanatory variables? a)
b)
c)
d)

(Short Answer)
4.9/5
(48)
SCENARIO 14-19
The marketing manager for a nationally franchised lawn service company would like to study the
characteristics that differentiate home owners who do and do not have a lawn service. A random
sample of 30 home owners located in a suburban area near a large city was selected; 11 did not have
a lawn service (code 0) and 19 had a lawn service (code 1). Additional information available
concerning these 30 home owners includes family income (Income, in thousands of dollars) and lawn
size (Lawn Size, in thousands of square feet).
The PHStat output is given below:
Binary Logistic Regression Predictor Coefficients SE Coef Z p -Value Intercept -7.8562 3.8224 -2.0553 0.0398 Income 0.0304 0.0133 2.2897 0.0220 Lawn Size 1.2804 0.6971 1.8368 0.0662 Deviance 25.3089
-Referring to Scenario 14-19, which of the following is the correct interpretation for the Lawn Size slope coefficient?
(Multiple Choice)
4.8/5
(29)
SCENARIO 14-17
Given below are results from the regression analysis where the dependent variable is the number of
weeks a worker is unemployed due to a layoff (Unemploy) and the independent variables are the age
of the worker (Age) and a dummy variable for management position (Manager: 1 = yes, 0 = no).
The results of the regression analysis are given below: \ Regression Statistics Multiple R 0.6391 R Square 0.4085 Adjusted R Square 0.3765 Standard Error 18.8929 Observations 40
Coefficients Standard Error t Stat P-value Intercept -0.2143 11.5796 -0.0185 0.9853 Age 1.4448 0.3160 4.5717 0.0000 Manager -22.5761 11.3488 -1.9893 0.0541
-Referring to Scenario 14-17, which of the following is a correct statement?

(Multiple Choice)
4.9/5
(36)
SCENARIO 14-16
What are the factors that determine the acceleration time (in sec.) from 0 to 60 miles per hour of a
car? Data on the following variables for 30 different vehicle models were collected: (Accel Time): Acceleration time in sec.
(Engine Size): c.c.
(Sedan): 1 if the vehicle model is a sedan and 0 otherwise
The regression results using acceleration time as the dependent variable and the remaining variables as the independent variables are presented below.
Regression Statistics Multiple R 0.6096 R Square 0.3716 Adjusted R Square 0.3251 Standard Error 1.4629 Observations 30
ANOVA
Coefficients Standard Error t Stat P-value Lower 95\% Upper 95\% Intercept 7.1052 0.6574 10.8086 0.0000 5.7564 8.4540 Engine Size -0.0005 0.0001 -3.6477 0.0011 -0.0008 -0.0002 Sedan 0.7264 0.5564 1.3056 0.2027 -0.4152 1.8681
-Referring to Scenario 14-16, what is the value of the test statistic to determine whether engine
size makes a significant contribution to the regression model in the presence of the other
independent variable at a 5% level of significance?

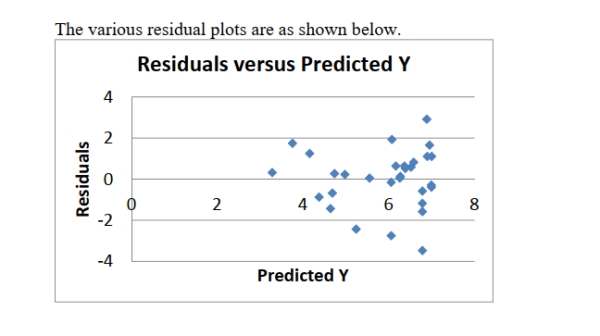
(Short Answer)
4.9/5
(34)
SCENARIO 14-17
Given below are results from the regression analysis where the dependent variable is the number of
weeks a worker is unemployed due to a layoff (Unemploy) and the independent variables are the age
of the worker (Age) and a dummy variable for management position (Manager: 1 = yes, 0 = no).
The results of the regression analysis are given below: \ Regression Statistics Multiple R 0.6391 R Square 0.4085 Adjusted R Square 0.3765 Standard Error 18.8929 Observations 40
Coefficients Standard Error t Stat P-value Intercept -0.2143 11.5796 -0.0185 0.9853 Age 1.4448 0.3160 4.5717 0.0000 Manager -22.5761 11.3488 -1.9893 0.0541
-Referring to Scenario 14-17, what is the standard error of estimate?

(Short Answer)
4.9/5
(39)
14-30 Introduction to Multiple Regression
-Referring to Scenario 14-7, the department head wants to test .
At a level of significance of 0.05, the null hypothesis is rejected.
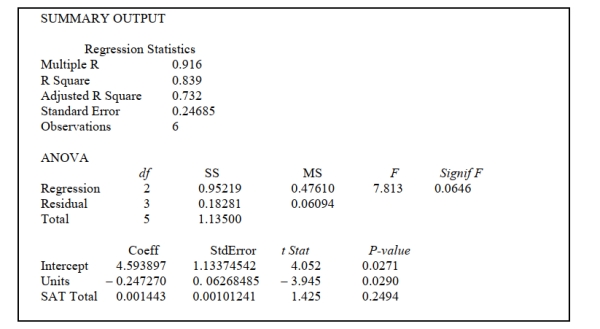
(True/False)
4.8/5
(31)
SCENARIO 14-8 A financial analyst wanted to examine the relationship between salary (in ) and 2 variables: age and experience in the field Exper). He took a sample of 20 employees and obtained the following Microsoft Excel output:
Regression Statistics Multiple R 0.8535 R Square 0.7284 Adjusted R Square 0.6964 Standard Error 10.5630 Observations 20
Coefficients Standard Error t Stat P-value Lower 95\% O5\% Intercept 1.5740 9.2723 0.1698 0.8672 -17.9888 21.1368 Age 1.3045 0.1956 6.6678 0.0000 0.8917 1.7173 Exper -0.1478 0.1944 -0.7604 0.4574 -0.5580 0.2624
Also the sum of squares due to the regression for the model that includes only Age is 5022.0654 while the
sum of squares due to the regression for the model that includes only Exper is 125.9848.
-Referring to Scenario 14-8, ____% of the variation in salary can be explained by the variation
in experience while holding age constant.

(Short Answer)
4.9/5
(36)
SCENARIO 14-15
The superintendent of a school district wanted to predict the percentage of students passing a sixth-
grade proficiency test. She obtained the data on percentage of students passing the proficiency test
(% Passing), mean teacher salary in thousands of dollars (Salaries), and instructional spending per
pupil in thousands of dollars (Spending) of 47 schools in the state. Following is the multiple regression output with Passing as the dependent variable,
Salaries and Spending:
Regression Statistics Multiple R 0.4276 R Square 0.1828 Adjusted R Square 0.1457 Standard Error 5.7351 Observations 47
ANOVA
Coefficients Standard Error t Stat \rho -value Lower 95\% Upper 95\% Intercept -72.9916 45.9106 -1.5899 0.1190 -165.5184 19.5352 Salary 2.7939 0.8974 3.1133 0.0032 0.9853 4.6025 Spending 0.3742 0.9782 0.3825 0.7039 -1.5972 2.3455
-Referring to Scenario 14-15, the alternative hypothesis implies that percentage of students passing the proficiency test is affected by both of the
explanatory variables.

(True/False)
4.9/5
(36)
SCENARIO 14-19
The marketing manager for a nationally franchised lawn service company would like to study the
characteristics that differentiate home owners who do and do not have a lawn service. A random
sample of 30 home owners located in a suburban area near a large city was selected; 11 did not have
a lawn service (code 0) and 19 had a lawn service (code 1). Additional information available
concerning these 30 home owners includes family income (Income, in thousands of dollars) and lawn
size (Lawn Size, in thousands of square feet).
The PHStat output is given below:
Binary Logistic Regression Predictor Coefficients SE Coef Z p -Value Intercept -7.8562 3.8224 -2.0553 0.0398 Income 0.0304 0.0133 2.2897 0.0220 Lawn Size 1.2804 0.6971 1.8368 0.0662 Deviance 25.3089
-Referring to Scenario 14-19, what should be the decision ('reject' or 'do not reject') on the null
hypothesis when testing whether LawnSize makes a significant contribution to the model in the
presence of Income at a 0.05 level of significance?
(Short Answer)
4.8/5
(35)
Showing 281 - 300 of 394
Filters
- Essay(0)
- Multiple Choice(0)
- Short Answer(0)
- True False(0)
- Matching(0)