Exam 7: Hypothesis Tests and Confidence Intervals in Multiple Regression
Exam 1: Economic Questions and Data17 Questions
Exam 2: Review of Probability70 Questions
Exam 3: Review of Statistics65 Questions
Exam 4: Linear Regression With One Regressor65 Questions
Exam 5: Regression With a Single Regressor: Hypothesis Tests and Confidence Intervals59 Questions
Exam 6: Linear Regression With Multiple Regressors65 Questions
Exam 7: Hypothesis Tests and Confidence Intervals in Multiple Regression64 Questions
Exam 8: Nonlinear Regression Functions63 Questions
Exam 9: Assessing Studies Based on Multiple Regression65 Questions
Exam 10: Regression With Panel Data50 Questions
Exam 11: Regression With a Binary Dependent Variable50 Questions
Exam 12: Instrumental Variables Regression50 Questions
Exam 13: Experiments and Quasi-Experiments50 Questions
Exam 14: Introduction to Time Series Regression and Forecasting50 Questions
Exam 15: Estimation of Dynamic Causal Effects50 Questions
Exam 16: Additional Topics in Time Series Regression50 Questions
Exam 17: The Theory of Linear Regression With One Regressor49 Questions
Exam 18: The Theory of Multiple Regression50 Questions
Select questions type
All of the following are examples of joint hypotheses on multiple regression coefficients, with the exception of
(Multiple Choice)
4.9/5
(35)
You have collected data from Major League Baseball (MLB)to find the determinants of winning. You have a general idea that both good pitching and strong hitting are needed to do well. However, you do not know how much each of these contributes separately. To investigate this problem, you collect data for all MLB during 1999 season. Your strategy is to first regress the winning percentage on pitching quality ("Team ERA"), second to regress the same variable on some measure of hitting ("OPS - On-base Plus Slugging percentage"), and third to regress the winning percentage on both.
Summary of the Distribution of Winning Percentage, On Base plus Slugging Percentage,
and Team Earned Run Average for MLB in 1999
The results are as follows: = 0.94 - 0.100 × teamera, R2 = 0.49, SER = 0.06.
(0.08)(0.017) = -0.68 + 1.513 × ops, R2=0.45, SER = 0.06.
(0.17)(0.221) = -0.19 - 0.099 × teamera + 1.490 × ops, R2=0.92, SER = 0.02.
(0.08)(0.008)(0.126)
(a)Use the t-statistic to test for the statistical significance of the coefficient.
(b)There are 30 teams in MLB. Does the small sample size worry you here when testing for significance?
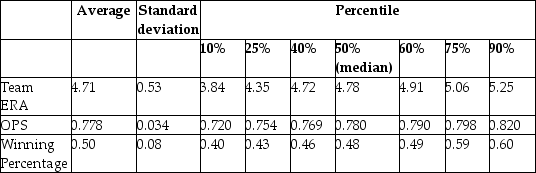
(Essay)
4.8/5
(33)
Using the California School data set from your textbook, you decide to run a regression of the average reading score (ScrRead)on the average mathematics score (ScrMaths). The result is as follows, where the numbers in parenthesis are homoskedasticity only standard errors: = 8.47 + 0.9895×ScrMaths
(13.20)(0.0202)
N = 420, R2 = 0.85, SER = 7.8
You believe that the average mathematics score is an unbiased predictor of the average reading score. Consider the above regression to be the unrestricted from which you would calculate SSRUnrestricted . How would you find the SSRRestricted? How many restrictions would have to impose?
(Essay)
4.9/5
(32)
Set up the null hypothesis and alternative hypothesis carefully for the following cases:
(a)k = 4, test for all coefficients other than the intercept to be zero
(b)k = 3, test for the slope coefficient of X1 to be unity, and the coefficients on the other explanatory variables to be zero
(c)k = 10, test for the slope coefficient of X1 to be zero, and for the slope coefficients of X2 and X3 to be the same but of opposite sign.
(d)k = 4, test for the slope coefficients to add up to unity
(Essay)
4.9/5
(28)
Let R2unrestricted and R2restricted be 0.4366 and 0.4149 respectively. The difference between the unrestricted and the restricted model is that you have imposed two restrictions. There are 420 observations. The F-statistic in this case is
(Multiple Choice)
4.7/5
(29)
The following linear hypothesis can be tested using the F-test with the exception of
(Multiple Choice)
4.8/5
(45)
To test joint linear hypotheses in the multiple regression model, you need to
(Multiple Choice)
4.9/5
(32)
The Solow growth model suggests that countries with identical saving rates and population growth rates should converge to the same per capita income level. This result has been extended to include investment in human capital (education)as well as investment in physical capital. This hypothesis is referred to as the "conditional convergence hypothesis," since the convergence is dependent on countries obtaining the same values in the driving variables. To test the hypothesis, you collect data from the Penn World Tables on the average annual growth rate of GDP per worker (g6090)for the 1960-1990 sample period, and regress it on the (i)initial starting level of GD-P per worker relative to the United States in 1960 (RelProd60), (ii)average population growth rate of the country (n), (iii)average investment share of GDP from 1960 to 1990 (SK - remember investment equals savings), and (iv)educational attainment in years for 1985 (Educ). The results for close to 100 countries is as follows (numbers in parentheses are for heteroskedasticity-robust standard errors): = 0.004 - 0.172 × n + 0.133 × SK + 0.002 × Educ - 0.044 × RelProd60,
(0.007)(0.209)(0.015)(0.001)(0.008)
R2=0.537, SER = 0.011
(a)Is the coefficient on this variable significantly different from zero at the 5% level? At the 1% level?
(b)Test for the significance of the other slope coefficients. Should you use a one-sided alternative hypothesis or a two-sided test? Will the decision for one or the other influence the decision about the significance of the parameters? Should you always eliminate variables which carry insignificant coefficients?
(Essay)
4.9/5
(35)
To calculate the homoskedasticity-only overall regression F-statistic, you need to compare the SSRrestricted with the SSRunrestricted. Consider the following output from a regression package, which reproduces the regression results of testscores on the student-teacher ratio, the percent of English learners, and the expenditures per student from your textbook:
Dependent Variable: TESTSCR
Method: Least Squares
Date: 07/30/06 Time: 17:55
Sample: 1 420
Included observations: 420
Sum of squared resid corresponds to SSRunrestricted. How are you going to find SSRrestricted?
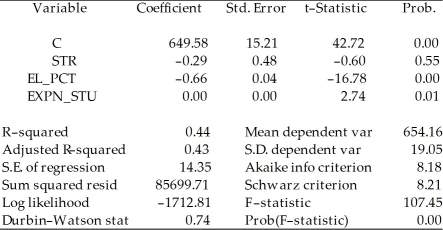
(Essay)
4.9/5
(37)
Analyzing a regression using data from a sub-sample of the Current Population Survey with about 4,000 observations, you realize that the regression R2, and the adjusted R2, 2, are almost identical. Why is that the case? In your textbook, you were told that the regression R2 will almost always increase when you add an explanatory variable, but that the adjusted measure does not have to increase with such an addition. Can this still be true?
(Essay)
4.8/5
(27)
All of the following are correct formulae for the homoskedasticity-only F-statistic, with the exception of
(Multiple Choice)
4.8/5
(44)
Explain carefully why testing joint hypotheses simultaneously, using the F-statistic, does not necessarily yield the same conclusion as testing them sequentially ("one at a time" method), using a series of t-statistics.
(Essay)
4.9/5
(32)
You have estimated the relationship between testscores and the student-teacher ratio under the assumption of homoskedasticity of the error terms. The regression output is as follows: = 698.9 - 2.28 × STR, and the standard error on the slope is 0.48. The homoskedasticity-only "overall" regression F- statistic for the hypothesis that the Regression R2 is zero is approximately
(Multiple Choice)
4.9/5
(34)
The confidence interval for a single coefficient in a multiple regression
(Multiple Choice)
4.7/5
(33)
Looking at formula (7.13)in your textbook for the homoskedasticity-only F-statistic,
give three conditions under which, ceteris paribus, you would find a large value, and hence would be likely to reject the null hypothesis.
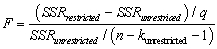
(Essay)
4.8/5
(40)
Consider the following two models to explain testscores.
Model 1:
Dependent Variable: TESTSCR
Method: Least Squares
Date: 07/31/06 Time: 17:52
Sample: 1 420
Included observations: 420
Model 2:
Dependent Variable: TESTSCR
Method: Least Squares
Date: 07/31/06 Time: 17:56
Sample: 1 420
Included observations: 420
Explain why you cannot use the F-test in this situation to discriminate between Model 1 and Model 2.
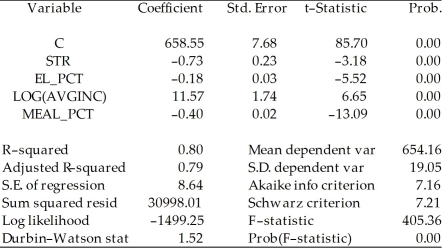
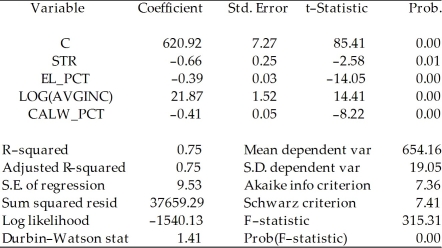
(Essay)
4.8/5
(35)
If you wanted to test, using a 5% significance level, whether or not a specific slope coefficient is equal to one, then you should
(Multiple Choice)
4.9/5
(28)
Consider the following regression using the California School data set from your textbook. = 681.44 - 0.61LchPct
n=420, R2=0.75, SER=9.45
where TestScore is the test score and LchPct is the percent of students eligible for subsidized lunch (average = 44.7, max = 100, min = 0).
a. What is the effect of a 20 percentage point increase in the student eligible for subsidized lunch?
b. Your textbook started with the following regression in Chapter 4: = 698.9 - 2.28STR
n=420, R2=0.051, SER=18.58
where STR is the student teacher ratio.
Your textbook tells you that in the multiple regression framework considered, the percentage of students eligible for subsidized lunch is a control variable, while the student teacher ratio is the variable of interest. Given that the regression R2 is so much higher for the first equation than for the second equation, shouldn't the role of the two variables be reversed? That is, shouldn't the student teacher ratio be the control variable while the percent of students eligible for subsidized lunch be the variable of interest?
(Essay)
4.9/5
(46)
The administration of your university/college is thinking about implementing a policy of coed floors only in dormitories. Currently there are only single gender floors. One reason behind such a policy might be to generate an atmosphere of better "understanding" between the sexes. The Dean of Students (DoS)has decided to investigate if such a behavior results in more "togetherness" by attempting to find the determinants of the gender composition at the dinner table in your main dining hall, and in that of a neighboring university, which only allows for coed floors in their dorms. The survey includes 176 students, 63 from your university/college, and 113 from a neighboring institution.
The Dean's first problem is how to define gender composition. To begin with, the survey excludes single persons' tables, since the study is to focus on group behavior. The Dean also eliminates sports teams from the analysis, since a large number of single-gender students will sit at the same table. Finally, the Dean decides to only analyze tables with three or more students, since she worries about "couples" distorting the results. The Dean finally settles for the following specification of the dependent variable:
GenderComp = Where " " stands for absolute value of Z. The variable can take on values from zero to fifty.
After considering various explanatory variables, the Dean settles for an initial list of eight, and estimates the following relationship, using heteroskedasticity-robust standard errors (this Dean obviously has taken an econometrics course earlier in her career and/or has an able research assistant): = 30.90 - 3.78 × Size - 8.81 × DCoed + 2.28 × DFemme +2.06 × DRoommate
(7.73)(0.63)(2.66)(2.42)(2.39)
- 0.17 × DAthlete + 1.49 × DCons - 0.81 SAT + 1.74 × SibOther, R2=0.24, SER = 15.50
(3.23)(1.10)(1.20)(1.43)
where Size is the number of persons at the table minus 3; DCoed is a binary variable, which takes on the value of 1 if you live on a coed floor; DFemme is a binary variable, which is 1 for females and zero otherwise; DRoommate is a binary variable which equals 1 if the person at the table has a roommate and is zero otherwise; DAthlete is a binary variable which is 1 if the person at the table is a member of an athletic varsity team; DCons is a variable which measures the political tendency of the person at the table on a seven-point scale, ranging from 1 being "liberal" to 7 being "conservative"; SAT is the SAT score of the person at the table measured on a seven-point scale, ranging from 1 for the category "900-1000" to 7 for the category "1510 and above"; and increasing by one for 100 point increases; and SibOther is the number of siblings from the opposite gender in the family the person at the table grew up with.
(a)Indicate which of the coefficients are statistically significant.
(b)Based on the above results, the Dean decides to specify a more parsimonious form by eliminating the least significant variables. Using the F-statistic for the null hypothesis that there is no relationship between the gender composition at the table and DFemme, DRoommate, DAthlete, and SAT, the regression package returns a value of 1.10. What are the degrees of freedom for the statistic? Look up the 1% and 5% critical values from the F- table and make a decision about the exclusion of these variables based on the critical values.
(c)The Dean decides to estimate the following specification next:
= 29.07 - 3.80 × Size - 9.75 × DCoed + 1.50 × DCons + 1.97 × SibOther,
(3.75)(0.62)(1.04)(1.04)(1.44)
R2=0.22 SER = 15.44
Calculate the t-statistics for the coefficients and discuss whether or not the Dean should attempt to simplify the specification further. Based on the results, what might some of the comments be that she will write up for the other senior administrators of your college? What are some of the potential flaws in her analysis? What other variables do you think she should have considered as explanatory factors?

(Essay)
4.9/5
(32)
Showing 21 - 40 of 64
Filters
- Essay(0)
- Multiple Choice(0)
- Short Answer(0)
- True False(0)
- Matching(0)