Exam 14: Introduction to Multiple Regression
Exam 1: Defining and Collecting Data207 Questions
Exam 2: Organizing and Visualizing Variables213 Questions
Exam 3: Numerical Descriptive Measures167 Questions
Exam 4: Basic Probability171 Questions
Exam 5: Discrete Probability Distributions217 Questions
Exam 6: The Normal Distributions and Other Continuous Distributions189 Questions
Exam 7: Sampling Distributions135 Questions
Exam 8: Confidence Interval Estimation189 Questions
Exam 9: Fundamentals of Hypothesis Testing: One-Sample Tests187 Questions
Exam 10: Two-Sample Tests208 Questions
Exam 11: Analysis of Variance216 Questions
Exam 12: Chi-Square and Nonparametric Tests178 Questions
Exam 13: Simple Linear Regression214 Questions
Exam 14: Introduction to Multiple Regression336 Questions
Exam 15: Multiple Regression Model Building99 Questions
Exam 16: Time-Series Forecasting173 Questions
Exam 17: Business Analytics115 Questions
Exam 18: A Roadmap for Analyzing Data329 Questions
Exam 19: Statistical Applications in Quality Management Online162 Questions
Exam 20: Decision Making Online129 Questions
Exam 21: Understanding Statistics: Descriptive and Inferential Techniques39 Questions
Select questions type
SCENARIO 14-16 What are the factors that determine the acceleration time (in sec.) from 0 to 60 miles per hour of a car? Data on the following variables for 30 different vehicle models were collected: Y (Accel Time): Acceleration time in sec. X₁ (Engine Size): c.c. X₂(Sedan): 1 if the vehicle model is a sedan and 0 otherwise The regression results using acceleration time as the dependent variable and the remaining variables as the independent variables are presented below.
The various residual plots are as shown below.
The coefficient of partial determinations
are 0.3301 and 0.0594 respectively. The coefficient of determination for the regression model using each of the 2 independent variables as the dependent variable and the other independent variable as independent variables
are, respectively, 0.0077 and 0.0077.
-Referring to Scenario 14-16, the 0 to 60 miles per hour acceleration time of a sedan is predicted to be 0.7264 seconds lower than that of a non-sedan with the same engine size.
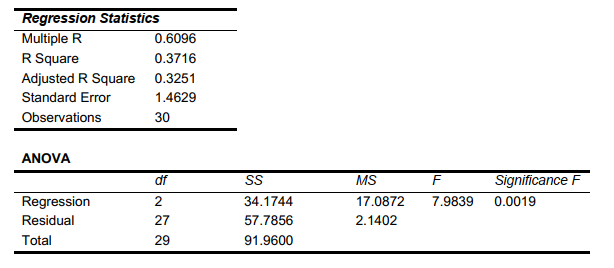

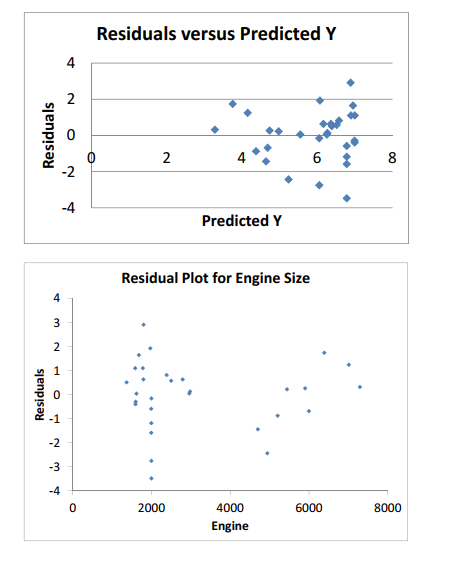
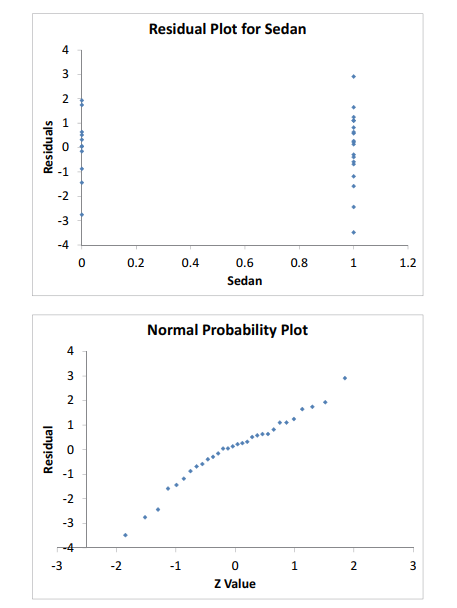


(True/False)
4.9/5
(30)
The interpretation of the slope is different in a multiple linear regression model as compared to a simple linear regression model.
(True/False)
4.7/5
(31)
If a categorical independent variable contains 2 categories, then _________ dummy variable(s)will be needed to uniquely represent these categories.
(Multiple Choice)
4.9/5
(28)
SCENARIO 14-11 A weight-loss clinic wants to use regression analysis to build a model for weight loss of a client (measured in pounds).Two variables thought to affect weight loss are client's length of time on the weight-loss program and time of session.These variables are described below: Y
Weight loss (in pounds)
Length of time in weight-loss program (in months)
1 if morning session, 0 if not Data for 25 clients on a weight-loss program at the clinic were collected and used to fit the interaction model: Y
Output from Microsoft Excel follows:
-Referring to Scenario 14-11, what null hypothesis would you test to determine whether the slope of the linear relationship between weight loss (Y)and time on the program
varies according to time of session?




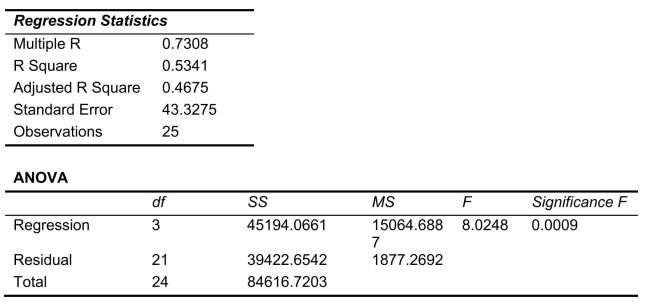


(Multiple Choice)
4.9/5
(35)
In a multiple regression problem involving two independent variables, if
is computed to be +2.0, it means that

(Multiple Choice)
4.9/5
(39)
The coefficient of multiple determination is calculated by taking the ratio of the regression sum of squares over the total sum of squares (SSR/SST)and subtracting that value from 1.
(True/False)
4.9/5
(33)
SCENARIO 14-11 A weight-loss clinic wants to use regression analysis to build a model for weight loss of a client (measured in pounds).Two variables thought to affect weight loss are client's length of time on the weight-loss program and time of session.These variables are described below: Y
Weight loss (in pounds)
Length of time in weight-loss program (in months)
1 if morning session, 0 if not Data for 25 clients on a weight-loss program at the clinic were collected and used to fit the interaction model: Y
Output from Microsoft Excel follows:
-Referring to Scenario 14-11, in terms of the
in the model, give the mean change in weight loss (Y)for every 1 month increase in time on the program
when attending the morning session.




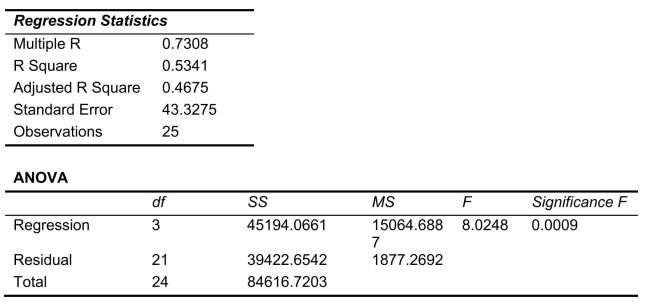



(Multiple Choice)
4.9/5
(44)
SCENARIO 14-3 An economist is interested to see how consumption for an economy (in $ billions)is influenced by gross domestic product ($ billions)and aggregate price (consumer price index).The Microsoft Excel output of this regression is partially reproduced below.
-Referring to Scenario 14-3, what is the estimated mean consumption level for an economy with GDP equal to $4 billion and an aggregate price index of 150?
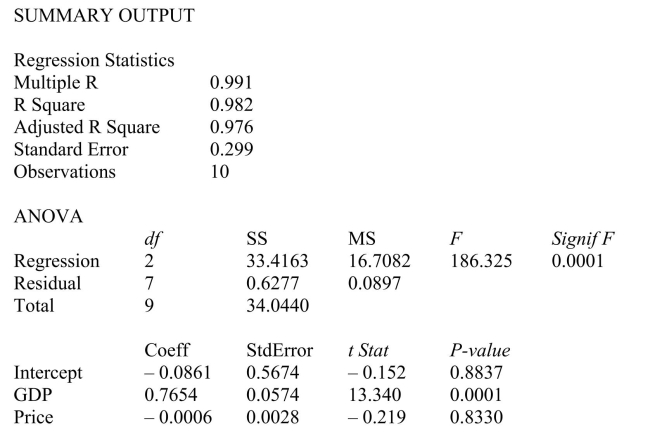
(Multiple Choice)
4.8/5
(40)
SCENARIO 14-5 A microeconomist wants to determine how corporate sales are influenced by capital and wage spending by companies.She proceeds to randomly select 26 large corporations and record information in millions of dollars.The Microsoft Excel output below shows results of this multiple regression.
-Referring to Scenario 14-5, one company in the sample had sales of $20 billion (Sales = 20,000).This company spent $300 million on capital and $700 million on wages. What is the residual (in millions of dollars)for this data point?
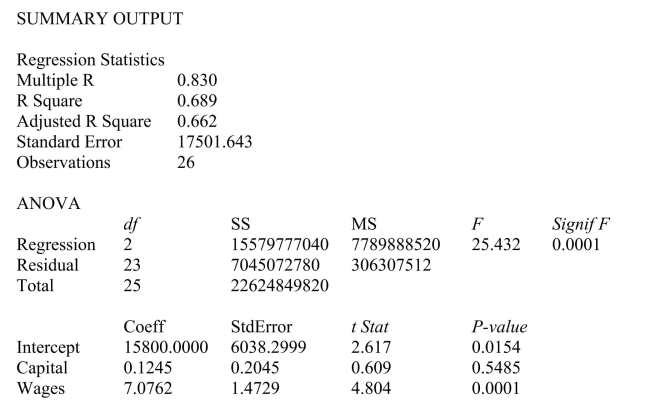
(Multiple Choice)
4.8/5
(31)
SCENARIO 14-17 Given below are results from the regression analysis where the dependent variable is the number of weeks a worker is unemployed due to a layoff (Unemploy)and the independent variables are the age of the worker (Age)and a dummy variable for management position (Manager: 1 = yes, 0 = no). The results of the regression analysis are given below:
-Referring to Scenario 14-17, what are the lower and upper limits of the 95% confidence interval estimate for the effect of a one year increase in age on the mean number of weeks a worker is unemployed due to a layoff after taking into consideration the effect of all the other independent variables?
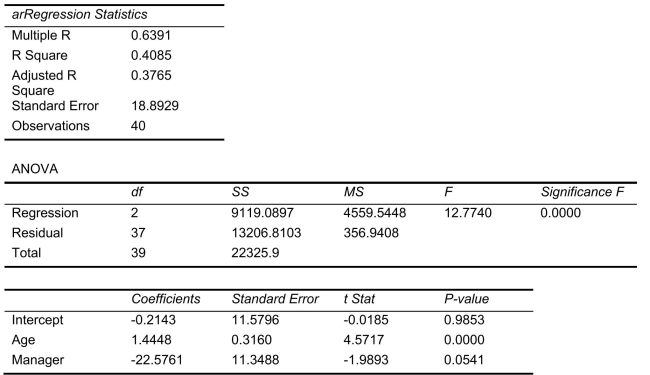
(Short Answer)
4.8/5
(36)
SCENARIO 14-10 You worked as an intern at We Always Win Car Insurance Company last summer.You notice that individual car insurance premiums depend very much on the age of the individual and the number of traffic tickets received by the individual.You performed a regression analysis in EXCEL and obtained the following partial information:
-Referring to Scenario 14-10, the 99% confidence interval for the change in mean insurance premiums of a person who has become 1 year older (i.e., the slope coefficient for AGE)- 1.4061
_______.
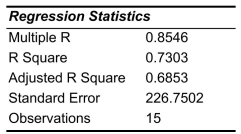
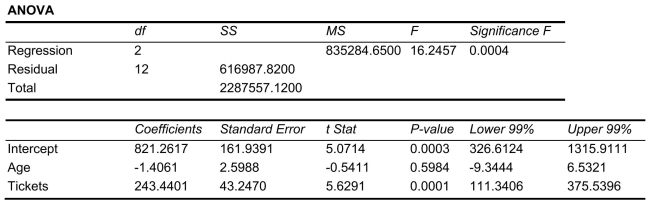

(Short Answer)
4.8/5
(39)
The slopes in a multiple regression model are called net regression coefficients.
(True/False)
4.8/5
(35)
SCENARIO 14-14 An automotive engineer would like to be able to predict automobile mileages.She believes that the two most important characteristics that affect mileage are horsepower and the number of cylinders (4 or 6)of a car.She believes that the appropriate model is
where
= horsepower
= 1 if 4 cylinders, 0 if 6 cylinders Y = mileage.
-Referring to Scenario 14-14, the fitted model for predicting mileages for 6-cylinder cars is ________.



(Multiple Choice)
4.9/5
(36)
SCENARIO 14-7 The department head of the accounting department wanted to see if she could predict the GPA of students using the number of course units and total SAT scores of each.She takes a sample of students and generates the following Microsoft Excel output:
-Referring to Scenario 14-7, the department head wants to test H₀:
= 0 .At a level of significance of 0.05, the null hypothesis is rejected.
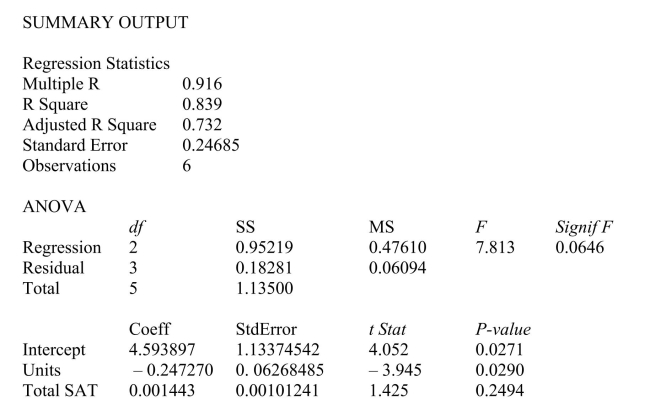

(True/False)
4.9/5
(32)
SCENARIO 14-6 One of the most common questions of prospective house buyers pertains to the cost of heating in dollars (Y).To provide its customers with information on that matter, a large real estate firm used the following 2 variables to predict heating costs: the daily minimum outside temperature in degrees of Fahrenheit
and the amount of insulation in inches
Given below is EXCEL output of the regression model.
-Referring to Scenario 14-6, what is your decision and conclusion for the test
level of significance?


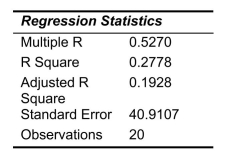
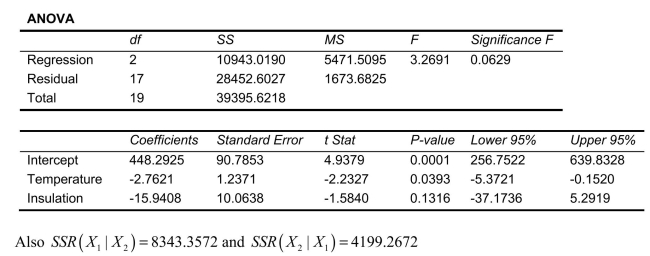

(Multiple Choice)
4.8/5
(39)
SCENARIO 14-4 A real estate builder wishes to determine how house size (House)is influenced by family income (Income)and family size (Size).House size is measured in hundreds of square feet and income is measured in thousands of dollars.The builder randomly selected 50 families and ran the multiple regression.Partial Microsoft Excel output is provided below:
-Referring to Scenario 14-4, what fraction of the variability in house size is explained by income and size of family?
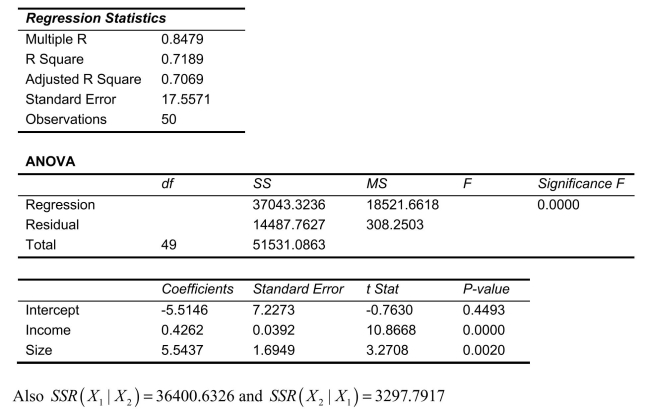
(Multiple Choice)
4.9/5
(42)
SCENARIO 14-17 Given below are results from the regression analysis where the dependent variable is the number of weeks a worker is unemployed due to a layoff (Unemploy)and the independent variables are the age of the worker (Age)and a dummy variable for management position (Manager: 1 = yes, 0 = no). The results of the regression analysis are given below:
-Referring to Scenario 14-17, the null hypothesis
implies that the number of weeks a worker is unemployed due to a layoff is not affected by any of the explanatory variables.
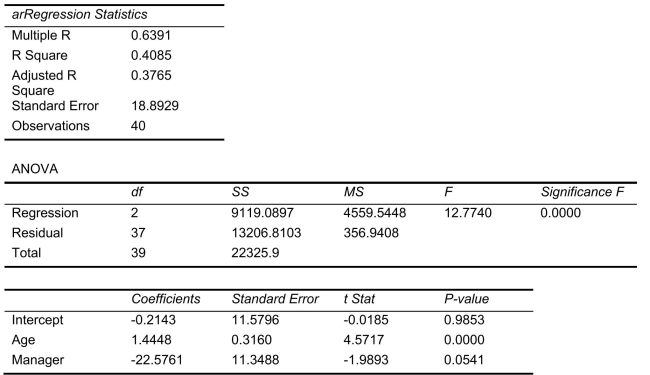
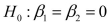
(True/False)
4.7/5
(41)
SCENARIO 14-8 A financial analyst wanted to examine the relationship between salary (in $1,000)and 2 variables: age
= Age)and experience in the field
= Exper).He took a sample of 20 employees and obtained the following Microsoft Excel output:
Also, the sum of squares due to the regression for the model that includes only Age is 5022.0654 while the sum of squares due to the regression for the model that includes only Exper is 125.9848.
-Referring to Scenario 14-8, the coefficient of partial determination
is ____.


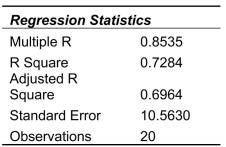
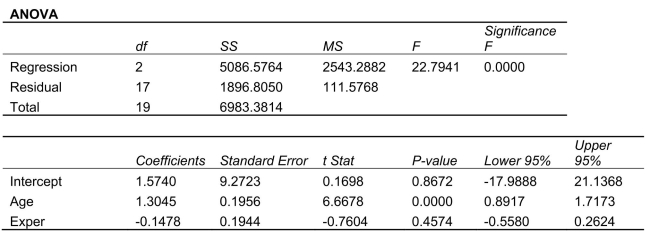

(Short Answer)
4.8/5
(32)
SCENARIO 14-10 You worked as an intern at We Always Win Car Insurance Company last summer.You notice that individual car insurance premiums depend very much on the age of the individual and the number of traffic tickets received by the individual.You performed a regression analysis in EXCEL and obtained the following partial information:
-Referring to Scenario 14-10, the regression sum of squares that is missing in the ANOVA table should be ______.
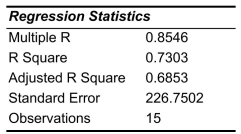
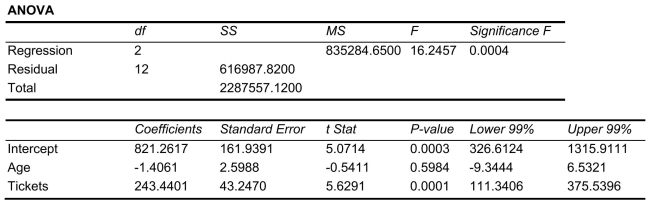
(Short Answer)
5.0/5
(36)
SCENARIO 14-16 What are the factors that determine the acceleration time (in sec.) from 0 to 60 miles per hour of a car? Data on the following variables for 30 different vehicle models were collected: Y (Accel Time): Acceleration time in sec. X₁ (Engine Size): c.c. X₂(Sedan): 1 if the vehicle model is a sedan and 0 otherwise The regression results using acceleration time as the dependent variable and the remaining variables as the independent variables are presented below.
The various residual plots are as shown below.
The coefficient of partial determinations
are 0.3301 and 0.0594 respectively. The coefficient of determination for the regression model using each of the 2 independent variables as the dependent variable and the other independent variable as independent variables
are, respectively, 0.0077 and 0.0077.
-Referring to Scenario 14-16, what is the p-value of the test statistic to determine whether engine size makes a significant contribution to the regression model in the presence of the other independent variable at a 5% level of significance?
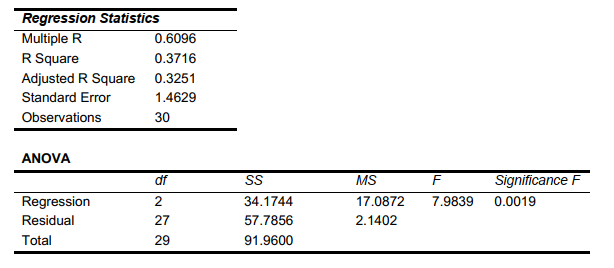

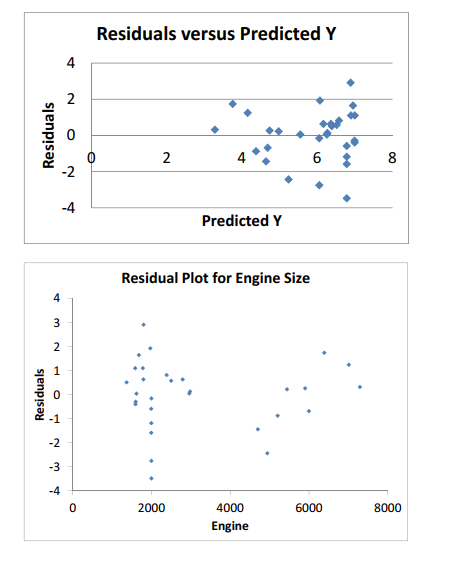
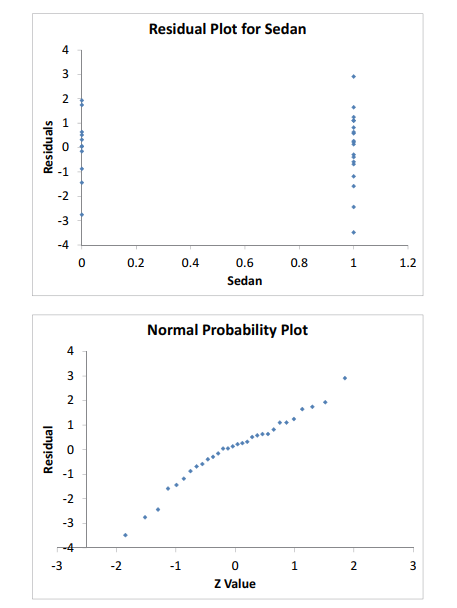


(Short Answer)
4.9/5
(43)
Showing 261 - 280 of 336
Filters
- Essay(0)
- Multiple Choice(0)
- Short Answer(0)
- True False(0)
- Matching(0)