Exam 14: Introduction to Multiple Regression
Exam 1: Defining and Collecting Data207 Questions
Exam 2: Organizing and Visualizing Variables213 Questions
Exam 3: Numerical Descriptive Measures167 Questions
Exam 4: Basic Probability171 Questions
Exam 5: Discrete Probability Distributions217 Questions
Exam 6: The Normal Distributions and Other Continuous Distributions189 Questions
Exam 7: Sampling Distributions135 Questions
Exam 8: Confidence Interval Estimation189 Questions
Exam 9: Fundamentals of Hypothesis Testing: One-Sample Tests187 Questions
Exam 10: Two-Sample Tests208 Questions
Exam 11: Analysis of Variance216 Questions
Exam 12: Chi-Square and Nonparametric Tests178 Questions
Exam 13: Simple Linear Regression214 Questions
Exam 14: Introduction to Multiple Regression336 Questions
Exam 15: Multiple Regression Model Building99 Questions
Exam 16: Time-Series Forecasting173 Questions
Exam 17: Business Analytics115 Questions
Exam 18: A Roadmap for Analyzing Data329 Questions
Exam 19: Statistical Applications in Quality Management Online162 Questions
Exam 20: Decision Making Online129 Questions
Exam 21: Understanding Statistics: Descriptive and Inferential Techniques39 Questions
Select questions type
SCENARIO 14-4 A real estate builder wishes to determine how house size (House)is influenced by family income (Income)and family size (Size).House size is measured in hundreds of square feet and income is measured in thousands of dollars.The builder randomly selected 50 families and ran the multiple regression.Partial Microsoft Excel output is provided below:
-Referring to Scenario 14-4, what annual income (in thousands of dollars)would an individual with a family size of 4 need to attain a predicted 10,000 square foot home (House = 100)?
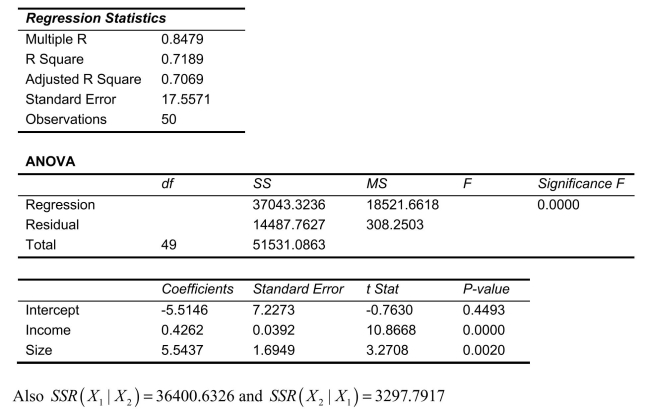
(Short Answer)
4.8/5
(39)
SCENARIO 14-18 A logistic regression model was estimated in order to predict the probability that a randomly chosen university or college would be a private university using information on mean total Scholastic Aptitude Test score (SAT)at the university or college and whether the TOEFL criterion is at least 90 (Toefl90 = 1 if yes, 0 otherwise.)The dependent variable, Y, is school type (Type = 1 if private and 0 otherwise).There are 80 universities in the sample. The PHStat output is given below:
-Referring to Scenario 14-18, which of the following is the correct interpretation for the SAT slope coefficient?
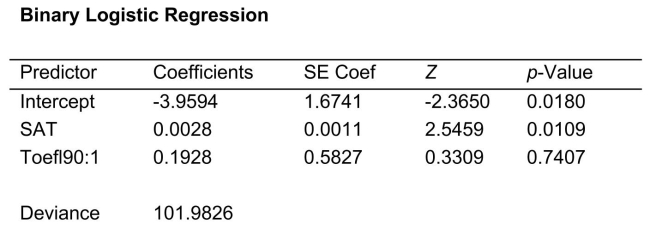
(Multiple Choice)
4.8/5
(33)
An interaction term in a multiple regression model may be used when
(Multiple Choice)
4.8/5
(41)
SCENARIO 14-2 A professor of industrial relations believes that an individual's wage rate at a factory (Y)depends on his performance rating
and the number of economics courses the employee successfully completed in college
The professor randomly selects 6 workers and collects the following information:
-Referring to Scenario 14-2, for these data, what is the estimated coefficient for performance rating, 


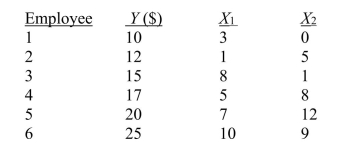

(Multiple Choice)
4.8/5
(37)
SCENARIO 14-9 You decide to predict gasoline prices in different cities and towns in the United States for your term project.Your dependent variable is price of gasoline per gallon and your explanatory variables are per capita income and the number of firms that manufacture automobile parts in and around the city.You collected data of 32 cities and obtained a regression sum of squares SSR= 122.8821.Your computed value of standard error of the estimate is 1.9549.
-Referring to Scenario 14-9, the value of adjusted
is

(Short Answer)
4.7/5
(30)
SCENARIO 14-5 A microeconomist wants to determine how corporate sales are influenced by capital and wage spending by companies.She proceeds to randomly select 26 large corporations and record information in millions of dollars.The Microsoft Excel output below shows results of this multiple regression.
-Referring to Scenario 14-5, what is the p-value for testing whether Wages have a negative impact on corporate sales?
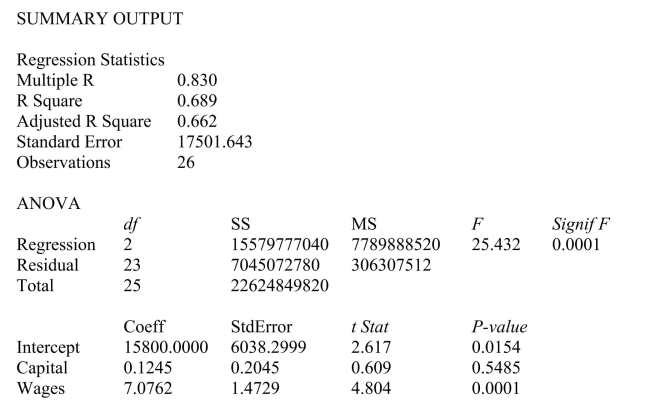
(Multiple Choice)
4.7/5
(30)
SCENARIO 14-19 The marketing manager for a nationally franchised lawn service company would like to study the characteristics that differentiate home owners who do and do not have a lawn service.A random sample of 30 home owners located in a suburban area near a large city was selected; 11 did not have a lawn service (code 0)and 19 had a lawn service (code 1).Additional information available concerning these 30 home owners includes family income (Income, in thousands of dollars)and lawn size (Lawn Size, in thousands of square feet). The PHStat output is given below:
-Referring to Scenario 14-19, what is the estimated probability that a home owner with a family income of $50,000 and a lawn size of 5,000 square feet will purchase a lawn service?
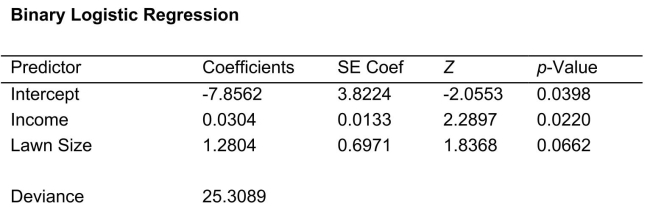
(Short Answer)
4.8/5
(39)
SCENARIO 14-15 The superintendent of a school district wanted to predict the percentage of students passing a sixth-grade proficiency test.She obtained the data on percentage of students passing the proficiency test (% Passing), mean teacher salary in thousands of dollars (Salaries), and instructional spending per pupil in thousands of dollars (Spending)of 47 schools in the state. Following is the multiple regression output with Y = % Passing as the dependent variable,
= Salaries and
Spending:
-Referring to Scenario 14-15, you can conclude definitively that mean teacher salary individually has no impact on the mean percentage of students passing the proficiency test, considering the effect of that instructional spending per pupil, at a 10% level of significance based solely on but not actually computing the 90% confidence interval estimate for 


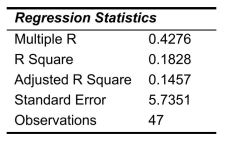
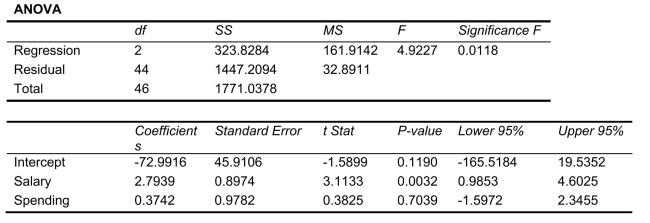

(True/False)
4.9/5
(36)
SCENARIO 14-16 What are the factors that determine the acceleration time (in sec.) from 0 to 60 miles per hour of a car? Data on the following variables for 30 different vehicle models were collected: Y (Accel Time): Acceleration time in sec. X₁ (Engine Size): c.c. X₂(Sedan): 1 if the vehicle model is a sedan and 0 otherwise The regression results using acceleration time as the dependent variable and the remaining variables as the independent variables are presented below.
The various residual plots are as shown below.
The coefficient of partial determinations
are 0.3301 and 0.0594 respectively. The coefficient of determination for the regression model using each of the 2 independent variables as the dependent variable and the other independent variable as independent variables
are, respectively, 0.0077 and 0.0077.
-Referring to Scenario 14-16, the 0 to 60 miles per hour acceleration time of a sedan is predicted to be 0.0005 seconds lower than that of a non-sedan with the same engine size.
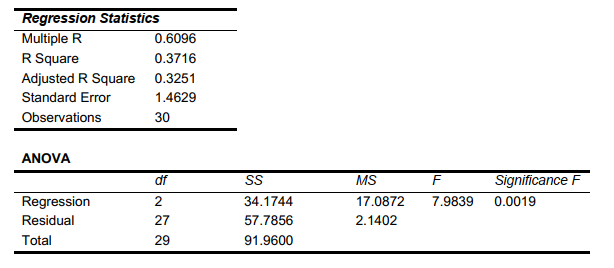

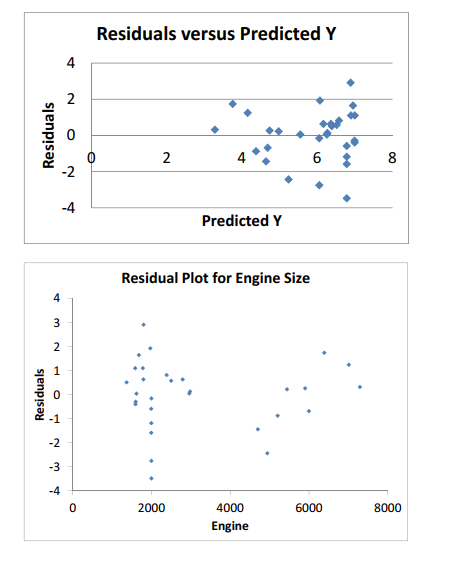
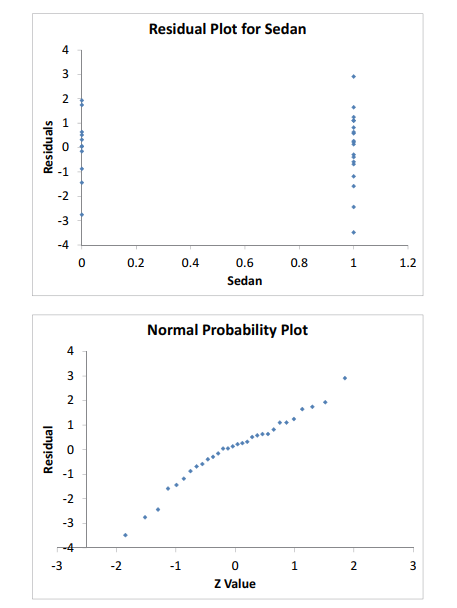


(True/False)
4.8/5
(32)
SCENARIO 14-5 A microeconomist wants to determine how corporate sales are influenced by capital and wage spending by companies.She proceeds to randomly select 26 large corporations and record information in millions of dollars.The Microsoft Excel output below shows results of this multiple regression.
-Referring to Scenario 14-5, what is the p-value for Capital?
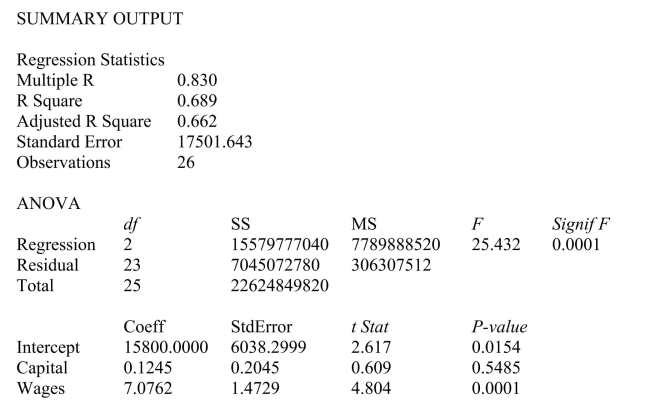
(Multiple Choice)
4.8/5
(32)
SCENARIO 14-18 A logistic regression model was estimated in order to predict the probability that a randomly chosen university or college would be a private university using information on mean total Scholastic Aptitude Test score (SAT)at the university or college and whether the TOEFL criterion is at least 90 (Toefl90 = 1 if yes, 0 otherwise.)The dependent variable, Y, is school type (Type = 1 if private and 0 otherwise).There are 80 universities in the sample. The PHStat output is given below:
-Referring to Scenario 14-18, the null hypothesis that the model is a good- fitting model cannot be rejected when allowing for a 5% probability of making a type I error.
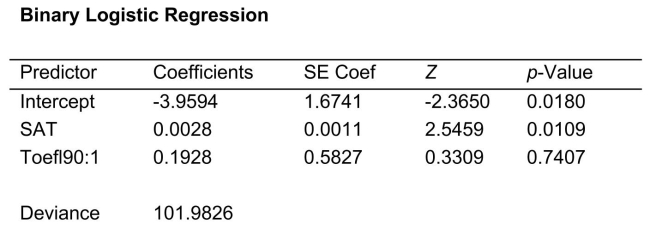
(True/False)
5.0/5
(33)
SCENARIO 14-15 The superintendent of a school district wanted to predict the percentage of students passing a sixth-grade proficiency test.She obtained the data on percentage of students passing the proficiency test (% Passing), mean teacher salary in thousands of dollars (Salaries), and instructional spending per pupil in thousands of dollars (Spending)of 47 schools in the state. Following is the multiple regression output with Y = % Passing as the dependent variable,
= Salaries and
Spending:
-Referring to Scenario 14-15, the null hypothesis
implies that percentage of students passing the proficiency test is not affected by either of the explanatory variables.


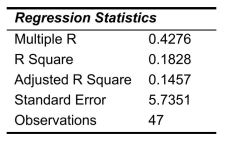
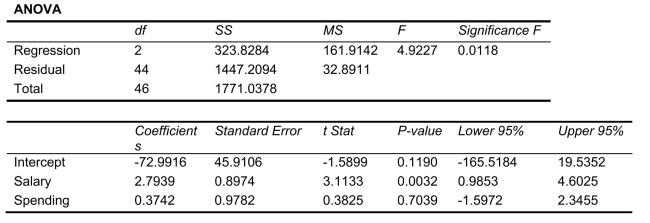
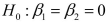
(True/False)
4.9/5
(29)
SCENARIO 14-15 The superintendent of a school district wanted to predict the percentage of students passing a sixth-grade proficiency test.She obtained the data on percentage of students passing the proficiency test (% Passing), mean teacher salary in thousands of dollars (Salaries), and instructional spending per pupil in thousands of dollars (Spending)of 47 schools in the state. Following is the multiple regression output with Y = % Passing as the dependent variable,
= Salaries and
Spending:
-Referring to Scenario 14-15, what is the p-value of the test statistic to determine whether there is a significant relationship between percentage of students passing the proficiency test and the entire set of explanatory variables?


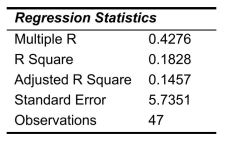
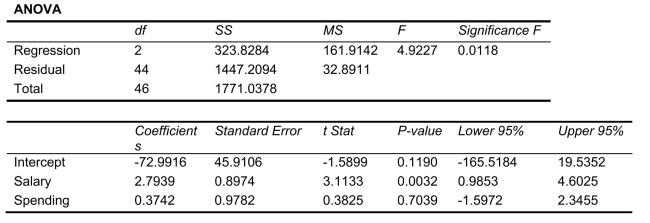
(Short Answer)
4.8/5
(34)
SCENARIO 14-7 The department head of the accounting department wanted to see if she could predict the GPA of students using the number of course units and total SAT scores of each.She takes a sample of students and generates the following Microsoft Excel output:
-Referring to Scenario 14-7, the department head decided to construct a 95% confidence interval for
.The confidence interval is from ________ to ________.
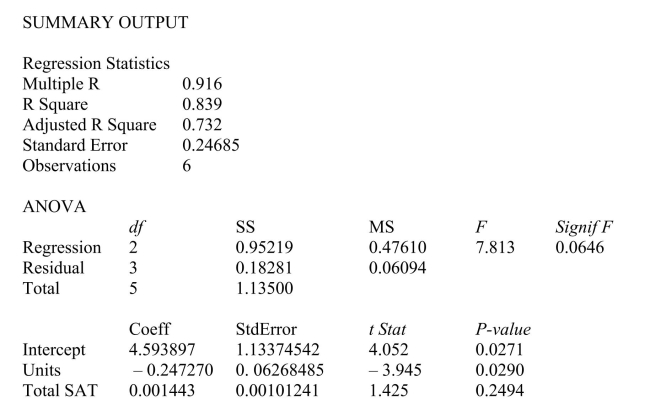

(Short Answer)
4.8/5
(32)
SCENARIO 14-15 The superintendent of a school district wanted to predict the percentage of students passing a sixth-grade proficiency test.She obtained the data on percentage of students passing the proficiency test (% Passing), mean teacher salary in thousands of dollars (Salaries), and instructional spending per pupil in thousands of dollars (Spending)of 47 schools in the state. Following is the multiple regression output with Y = % Passing as the dependent variable,
= Salaries and
Spending:
-Referring to Scenario 14-15, there is sufficient evidence that the percentage of students passing the proficiency test depends on at least one of the explanatory variables at a 5% level of significance.


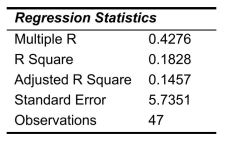
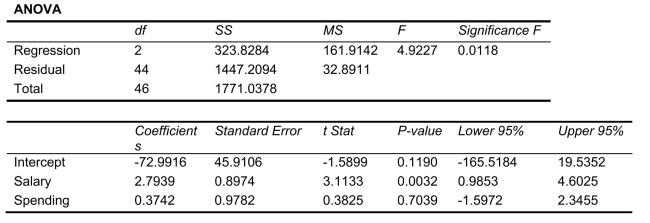
(True/False)
4.8/5
(38)
When an additional explanatory variable is introduced into a multiple regression model, the adjusted
can never decrease.

(True/False)
4.8/5
(29)
SCENARIO 14-5 A microeconomist wants to determine how corporate sales are influenced by capital and wage spending by companies.She proceeds to randomly select 26 large corporations and record information in millions of dollars.The Microsoft Excel output below shows results of this multiple regression.
-Referring to Scenario 14-5, one company in the sample had sales of $21.439 billion (Sales = 21,439).This company spent $300 million on capital and $700 million on wages. What is the residual (in millions of dollars)for this data point?
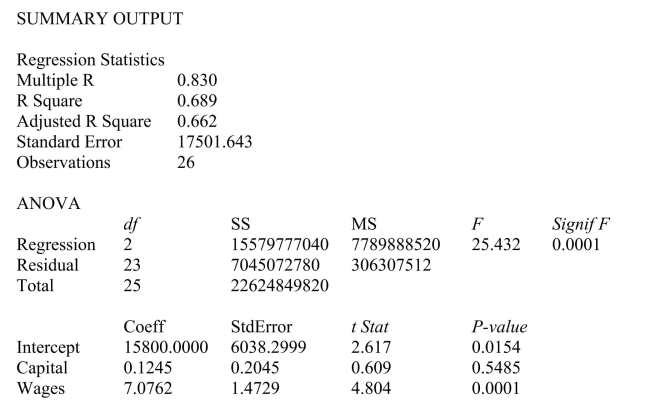
(Multiple Choice)
4.8/5
(29)
SCENARIO 14-4 A real estate builder wishes to determine how house size (House)is influenced by family income (Income)and family size (Size).House size is measured in hundreds of square feet and income is measured in thousands of dollars.The builder randomly selected 50 families and ran the multiple regression.Partial Microsoft Excel output is provided below:
-Referring to Scenario 14-4, which of the following values for the level of significance is the smallest for which the regression model as a whole is significant?
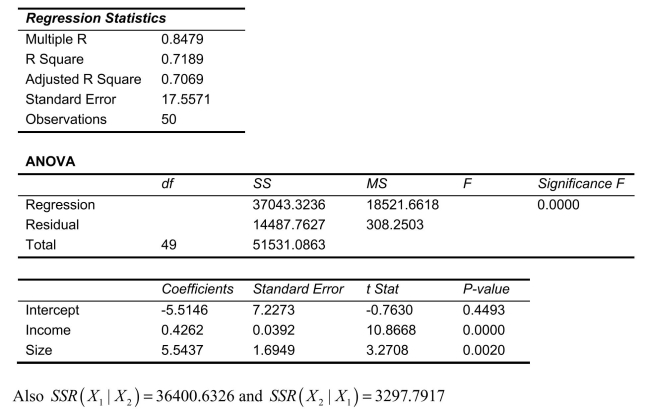
(Multiple Choice)
4.9/5
(33)
SCENARIO 14-4 A real estate builder wishes to determine how house size (House)is influenced by family income (Income)and family size (Size).House size is measured in hundreds of square feet and income is measured in thousands of dollars.The builder randomly selected 50 families and ran the multiple regression.Partial Microsoft Excel output is provided below:
-Referring to Scenario 14-4, the value of the partial F test statistic is ____ for H₀ : Variable
does not significantly improve the model after variable
has been included H₁ : Variable
significantly improves the model after variable
has been included
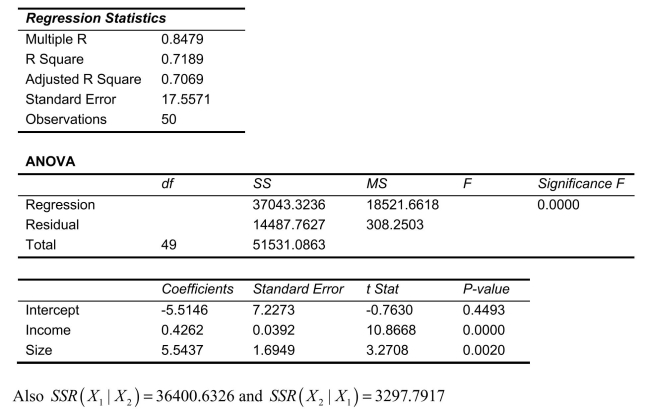




(Short Answer)
4.8/5
(24)
SCENARIO 14-5 A microeconomist wants to determine how corporate sales are influenced by capital and wage spending by companies.She proceeds to randomly select 26 large corporations and record information in millions of dollars.The Microsoft Excel output below shows results of this multiple regression.
-Referring to Scenario 14-5, what is the p-value for Wages?
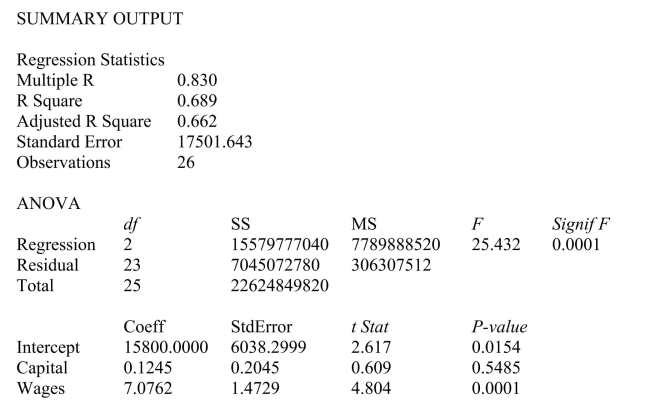
(Multiple Choice)
4.9/5
(32)
Showing 41 - 60 of 336
Filters
- Essay(0)
- Multiple Choice(0)
- Short Answer(0)
- True False(0)
- Matching(0)