Exam 17: Multiple Regression
Exam 1: What Is Statistics39 Questions
Exam 2: Graphical and Tabular Descriptive Techniques192 Questions
Exam 3: Numerical Descriptive Techniques215 Questions
Exam 4: Data Collection and Sampling82 Questions
Exam 5: Probability200 Questions
Exam 6: Random Variables and Discrete Probability Distributions158 Questions
Exam 7: Continuous Probability Distributions149 Questions
Exam 8: Sampling Distributions127 Questions
Exam 9: Introduction to Estimation85 Questions
Exam 10: Introduction to Hypothesis Testing178 Questions
Exam 11: Inference About a Population75 Questions
Exam 12: Inference About Comparing Two Populations, Part 183 Questions
Exam 13: Inference About Comparing Two Populations, Part 284 Questions
Exam 14: Analysis of Variance125 Questions
Exam 15: Chi-Squared Tests118 Questions
Exam 16: Simple Linear Regression and Correlation231 Questions
Exam 17: Multiple Regression143 Questions
Exam 18: Review of Statistical Inference182 Questions
Select questions type
A multiple regression model has the form:
. As x2 increases by one unit, holding x1 constant, then the value of y will increase by:

(Multiple Choice)
4.8/5
(33)
To test the validity of a multiple regression model, we test the null hypothesis that the regression coefficients are all zero by applying the:
(Multiple Choice)
4.7/5
(42)
A multiple regression model has the form
. The coefficient b1 is interpreted as the average change in y per unit change in x1.

(True/False)
4.8/5
(41)
Student's Final Grade: A statistics professor investigated some of the factors that affect an individual student's final grade in her course. She proposed the multiple regression model
, where y is the final grade (out of 100 points), x1 is the number of lectures skipped, x2 is the number of late assignments, and x3 is the midterm exam score (out of 100). The professor recorded the data for 50 randomly selected students. The computer output is shown below.
THE REGRESSION EQUATION IS
ANALYSIS OF VARIANCE
-Interpret the coefficient b1.


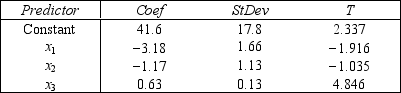


(Essay)
4.9/5
(34)
Life Expectancy
An actuary wanted to develop a model to predict how long individuals will live. After consulting a number of physicians, she collected the age at death (y), the average number of hours of exercise per week (x1), the cholesterol level (x2), and the number of points that the individual's blood pressure exceeded the recommended value (x3). A random sample of 40 individuals was selected. The computer output of the multiple regression model is shown below.
THE REGRESSION EQUATION IS
y = 55.8 + 1.79x1 -0.021x2 - 0.061x3
ANALYSIS OF VARIANCE
-{Life Expectancy Narrative} Is there sufficient evidence at the 5% significance level to infer that the number of points that the individual's blood pressure exceeded the recommended value and the age at death are negatively linearly related?
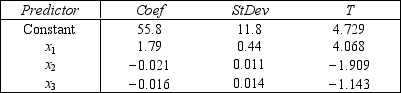


(Essay)
4.7/5
(36)
The coefficient of determination R2 measures the proportion of variation in y that is explained by the explanatory variables included in the model.
(True/False)
4.9/5
(44)
In reference to the equation
, the value 0.12 is the average change in y per unit change in x1, when x2 is held constant.

(True/False)
4.7/5
(34)
In testing the significance of a multiple regression model with three independent variables, the null hypothesis is
.

(True/False)
4.9/5
(31)
In a multiple regression analysis involving 40 observations and 5 independent variables, the following statistics are given: Total variation in y = 350 and SSE = 50. Then, the coefficient of determination is:
(Multiple Choice)
4.8/5
(36)
A multiple regression equation has a coefficient of determination of 0.81. Then, the percentage of the variation in y that is explained by the regression equation is 90%.
(True/False)
4.8/5
(33)
A multiple regression model is assessed to be good if the error sum of squares SSE and the standard error of estimate s are both small, the coefficient of determination R2 is close to 1, and the value of the test statistic F is large.
(True/False)
4.9/5
(29)
A multiple regression model involves 10 independent variables and 30 observations. If we want to test at the 5% significance level whether one of the coefficients is = 0 (vs. 0) the critical value will be:
(Multiple Choice)
4.9/5
(32)
The validity of a multiple regression model is tested using a(n) _________ test.
(Short Answer)
4.8/5
(29)
One of the consequences of multicollinearity in multiple regression is inflated standard errors in some or all of the estimated slope coefficients.
(True/False)
4.8/5
(33)
A high correlation between two independent variables is an indication of ____________________.
(Short Answer)
4.8/5
(35)
Multicollinearity is present if the dependent variable is linearly related to one of the explanatory variables.
(True/False)
4.8/5
(29)
For a multiple regression model, the following statistics are given: Total variation in y = 500, SSE = 80, and n = 25. Then, the coefficient of determination is:
(Multiple Choice)
4.9/5
(28)
If a group of independent variables are not significant individually but are significant as a group at a specified level of significance, this is most likely due to:
(Multiple Choice)
4.9/5
(32)
Showing 61 - 80 of 143
Filters
- Essay(0)
- Multiple Choice(0)
- Short Answer(0)
- True False(0)
- Matching(0)