Exam 16: Regression Analysis: Model Building
Exam 1: Data and Statistics98 Questions
Exam 2: Descriptive Statistics: Tabular and Graphical Displays62 Questions
Exam 3: Descriptive Statistics: Numerical Measures173 Questions
Exam 4: Introduction to Probability138 Questions
Exam 5: Discrete Probability Distributions123 Questions
Exam 6: Continuous Probability Distributions174 Questions
Exam 7: Sampling and Sampling Distributions133 Questions
Exam 8: Interval Estimation137 Questions
Exam 9: Hypothesis Tests148 Questions
Exam 10: Inference About Means and Proportions With Two Populations121 Questions
Exam 11: Inferences About Population Variances90 Questions
Exam 12: Comparing Multiple Proportions, Test of Independence and Goodness of Fit90 Questions
Exam 13: Experimental Design and Analysis of Variance115 Questions
Exam 14: Simple Linear Regression146 Questions
Exam 15: Multiple Regression115 Questions
Exam 16: Regression Analysis: Model Building76 Questions
Exam 17: Time Series Analysis and Forecasting68 Questions
Exam 18: Nonparametric Methods81 Questions
Exam 19: Statistical Methods for Quality Control29 Questions
Exam 20: Index Numbers52 Questions
Exam 21: Decision Analysis on Website65 Questions
Exam 22: Sample Survey on Website63 Questions
Select questions type
In a regression analysis involving 20 observations and five independent variables, the following information was obtained.
Fill in all the blanks in the above ANOVA table.
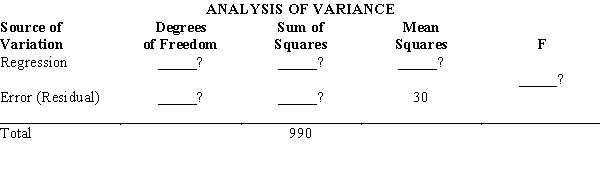
(Essay)
4.9/5
(32)
We are interested in determining what type of model best describes the relationship between two variables x and y.
a.For a given data set, an estimated regression equation relating x and y of the form
was developed, using Excel. The results are shown below. Comment on the adequacy of this equation for predicting y. Let = .05.
b.An estimated regression equation for the same data set (as in part a) of the form
was developed. The Excel output is shown below. Comment on the adequacy of this equation for predicting y. Let = .05.
c.Use the results of Part b and predict y when x = 4.

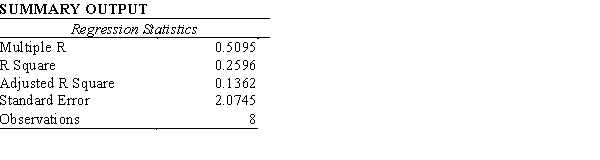
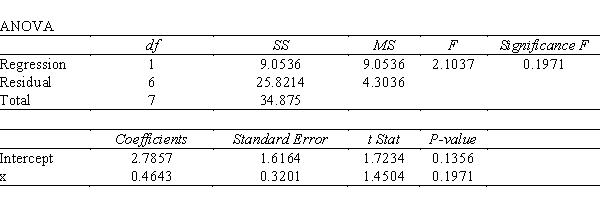

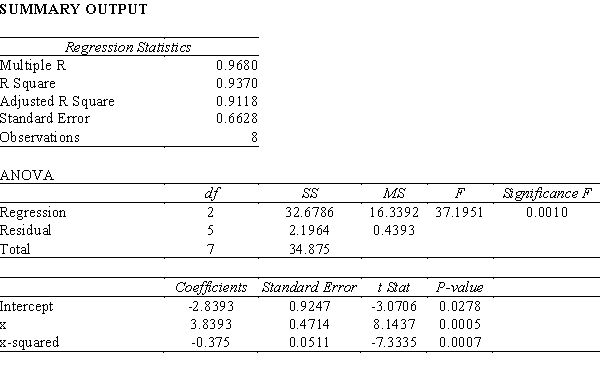
(Essay)
4.9/5
(34)
Exhibit 16-1
In a regression analysis involving 25 observations, the following estimated regression equation was developed.
Also, the following standard errors and the sum of squares were obtained.
Sb1 = 3
Sb2 = 6
Sb3 = 7
SST = 4,800
SSE = 1,296
-Refer to Exhibit 16-1. The coefficient of X2

(Multiple Choice)
4.7/5
(31)
A test used to determine whether or not first order autocorrelation is present is
(Multiple Choice)
4.9/5
(34)
Exhibit 16-1
In a regression analysis involving 25 observations, the following estimated regression equation was developed.
Also, the following standard errors and the sum of squares were obtained.
Sb1 = 3
Sb2 = 6
Sb3 = 7
SST = 4,800
SSE = 1,296
-Refer to Exhibit 16-1. The coefficient of X1

(Multiple Choice)
4.7/5
(37)
Exhibit 16-1
In a regression analysis involving 25 observations, the following estimated regression equation was developed.
Also, the following standard errors and the sum of squares were obtained.
Sb1 = 3
Sb2 = 6
Sb3 = 7
SST = 4,800
SSE = 1,296
-Refer to Exhibit 16-1. The coefficient of X3

(Multiple Choice)
4.9/5
(40)
Exhibit 16-4
In a laboratory experiment, data were gathered on the life span (Y in months) of 33 rats, units of daily protein intake (X1), and whether or not agent X2 (a proposed life extending agent) was added to the rats diet (X2 = 0 if agent X2 was not added, and X2 = 1 if agent was added.) From the results of the experiment, the following regression model was developed.
Also provided are SSR = 60 and SST = 180.
-Refer to Exhibit 16-4. If we want to test for the significance of the model, the critical value of F at 95% confidence is

(Multiple Choice)
4.9/5
(33)
When a regression model was developed relating sales (Y) of a company to its product's price (X1), the SSE was determined to be 495. A second regression model relating sales (Y) to product's price (X1) and competitor's product price (X2) resulted in an SSE of 396. At = 0.05, determine if the competitor's product's price contributed significantly to the model. The sample size for both models was 33.
(Essay)
4.7/5
(27)
Exhibit 16-1
In a regression analysis involving 25 observations, the following estimated regression equation was developed.
Also, the following standard errors and the sum of squares were obtained.
Sb1 = 3
Sb2 = 6
Sb3 = 7
SST = 4,800
SSE = 1,296
-Refer to Exhibit 16-1. The multiple coefficient of determination is

(Multiple Choice)
4.7/5
(41)
Exhibit 16-4
In a laboratory experiment, data were gathered on the life span (Y in months) of 33 rats, units of daily protein intake (X1), and whether or not agent X2 (a proposed life extending agent) was added to the rats diet (X2 = 0 if agent X2 was not added, and X2 = 1 if agent was added.) From the results of the experiment, the following regression model was developed.
Also provided are SSR = 60 and SST = 180.
-Refer to Exhibit 16-4. The test statistic for testing the significance of the model is

(Multiple Choice)
4.8/5
(39)
The following are partial results of a regression analysis involving sales (Y in millions of dollars), advertising expenditures (X1 in thousands of dollars), and number of salespeople (X2) for a corporation. The regression was performed on a sample of 10 observations.
a.At = 0.05, test for the significance of the coefficient of advertising.
b.If the company uses $20,000 in advertisement and has 300 salespersons, what are the expected sales? (Give your answer in dollars.)

(Essay)
4.9/5
(38)
The joint effect of two variables acting together is called
(Multiple Choice)
4.8/5
(38)
Exhibit 16-4
In a laboratory experiment, data were gathered on the life span (Y in months) of 33 rats, units of daily protein intake (X1), and whether or not agent X2 (a proposed life extending agent) was added to the rats diet (X2 = 0 if agent X2 was not added, and X2 = 1 if agent was added.) From the results of the experiment, the following regression model was developed.
Also provided are SSR = 60 and SST = 180.
-Refer to Exhibit 16-4. The life expectancy of a rat that was given 2 units of agent X2 daily, but was not given any protein is

(Multiple Choice)
4.9/5
(39)
The correlation in error terms that arises when the error terms at successive points in time are related is termed
(Multiple Choice)
4.9/5
(28)
The following estimated regression equation has been developed for the relationship between y, the dependent variable, and x, the independent variable.
The sample size for this regression model was 23, and SSR = 600 and SSE = 400.
a.Compute the coefficient of determination.
b.Using = .05, test for a significant relationship.

(Essay)
4.8/5
(43)
A regression analysis was applied in order to determine the relationship between a dependent variable and 4 independent variables. The following information was obtained from the regression analysis.
R Square = 0.80
SSR = 680
Total number of observations n = 45
a.Fill in the blanks in the following ANOVA table.
b.At = 0.05 level of significance, test to determine if the model is significant.
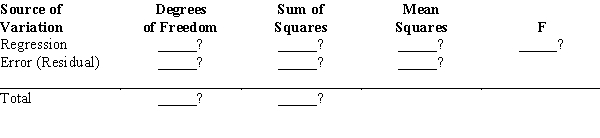
(Essay)
4.9/5
(28)
In a regression analysis involving 18 observations and four independent variables, the following information was obtained.
Based on the above information, fill in all the blanks in the following ANOVA table. 


(Essay)
4.7/5
(33)
In a regression analysis involving 21 observations and 4 independent variables, the following information was obtained.
= 0.80
S = 5.0
Based on the above information, fill in all the blanks in the following ANOVa.Hint:
=
, but also
= 1-
. 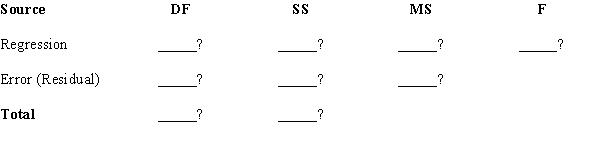





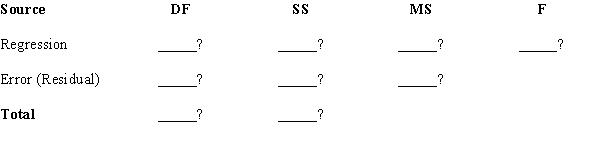
(Essay)
4.8/5
(36)
Showing 21 - 40 of 76
Filters
- Essay(0)
- Multiple Choice(0)
- Short Answer(0)
- True False(0)
- Matching(0)